Documents
Presentation Slides
LFZip: Lossy Compression of Multivariate Floating-Point Time Series Data via Improved Prediction
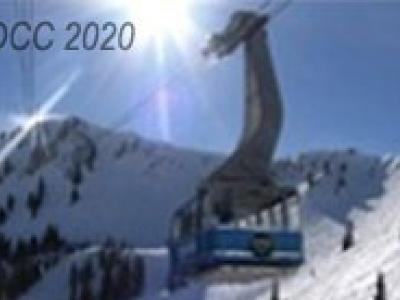
- Citation Author(s):
- Submitted by:
- Shubham Chandak
- Last updated:
- 18 March 2020 - 5:02pm
- Document Type:
- Presentation Slides
- Document Year:
- 2020
- Event:
- Presenters:
- Shubham Chandak
- Paper Code:
- 111
- Categories:
- Log in to post comments
Time series data compression is emerging as an important problem with the growth in IoT devices and sensors. Due to the presence of noise in these datasets, lossy compression can often provide significant compression gains without impacting the performance of downstream applications. In this work, we propose an error-bounded lossy compressor, LFZip, for multivariate floating-point time series data that provides guaranteed reconstruction up to user-specified maximum absolute error. The compressor is based on the prediction-quantization-entropy coder framework and benefits from improved prediction using linear models and neural networks. We evaluate the compressor on several time series datasets where it outperforms the existing state-of-the-art error-bounded lossy compressors. The code and data are available at https://github.com/shubhamchandak94/LFZip.
slides.pdf
