Documents
Poster
Deep Clustering of Compressed Variational Embeddings
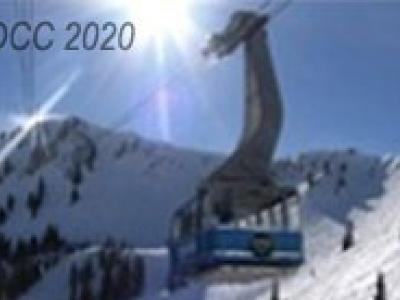
- Citation Author(s):
- Submitted by:
- Suya Wu
- Last updated:
- 28 March 2020 - 11:30pm
- Document Type:
- Poster
- Document Year:
- 2020
- Event:
- Presenters:
- Suya Wu
- Categories:
- Log in to post comments
Motivated by the ever-increasing demands for limited communication bandwidth and low-power consumption, we propose a new methodology, named joint Variational Autoencoders with Bernoulli mixture models (VAB), for performing clustering in the compressed data domain. The idea is to reduce the data dimension by Variational Autoencoders (VAEs) and group data representations by Bernoulli mixture models (BMMs). Once jointly trained for compression and clustering, the model can be decomposed into two parts: a data vendor that encodes the raw data into compressed data, and a data consumer that classifies the received (compressed) data. In this way, the data vendor benefits from data security and communication bandwidth, while the data consumer benefits from low computational complexity. To enable training using the gradient descent algorithm, we propose to use the Gumbel-Softmax distribution to resolve the infeasibility of the back-propagation algorithm when assessing categorical samples.