Documents
Poster
Fast CU Size Decision Using Machine Learning for Depth Map Coding in 3D-HEVC
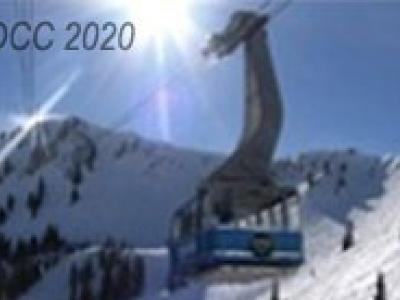
- Citation Author(s):
- Submitted by:
- Ruyi Zhang
- Last updated:
- 29 March 2020 - 10:57pm
- Document Type:
- Poster
- Document Year:
- 2020
- Event:
- Paper Code:
- 106
- Categories:
- Log in to post comments
3D-High Efficiency Video Coding (3D-HEVC) is a video compression standard developed for multi-view video plus depth map coding based on the latest HEVC coding standard. We propose an eXtreme Gradient Boosting (XGBoost) system based fast coding unit (CU) level decision for depth maps, which is used to solve the problem of high coding complexity caused by the addition of depth maps and new coding tools in 3D-HEVC. We explore the application of data mining and machine learning in video coding by using texture feature attributes that are highly correlated with CU size. The algorithm is mainly divided into three parts as shown in Figure 1. The algorithm comprises two parts: Models training and fast CU segmentation decision. In the first part, we use data mining and machine learning to construct the decision models by using the texture information of the depth maps as the feature attribute vectors and whether the current CU continues to be divided into sub-CUs as class labels. In the second part, feature attributes were extracted from the coding process, and the trained models were used to decide if the CU continues to partition. Experimental results demonstrated that proposed algorithm yields average 43.52% encoding time reduction with 0.12% BD-rate decrease on V/T and 0.37% increase on S/T under the all intra configuration, compared with the reference software HTM-16.0. In addition, compared with related work, the proposed method achieves different degrees of improvement in coding performance.