Documents
Presentation Slides
Unsupervised Domain Adaptation for Semantic Segmentation with Symmetric Adaptation Consistency
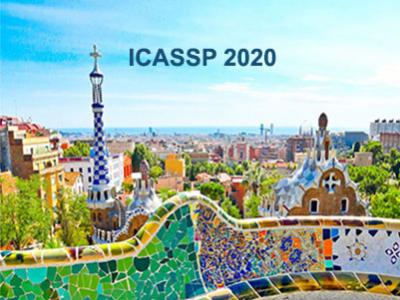
- Citation Author(s):
- Submitted by:
- Zongyao Li
- Last updated:
- 14 May 2020 - 3:42am
- Document Type:
- Presentation Slides
- Document Year:
- 2020
- Event:
- Presenters:
- Zongyao Li
- Paper Code:
- 4631
- Categories:
- Log in to post comments
Unsupervised domain adaptation, which leverages label information from other domains to solve tasks on a domain without any labels, can alleviate the problem of the scarcity of labels and expensive labeling costs faced by supervised semantic segmentation. In this paper, we utilize adversarial learning and semi-supervised learning simultaneously to solve the task of unsupervised domain adaptation in semantic segmentation. We propose a new approach that trains two segmentation models with the adversarial learning symmetrically and further introduces the consistency between the outputs of the two models into the semi-supervised learning to improve the accuracy of pseudo labels which significantly affect the final adaptation performance. We achieve state-of-the-art semantic segmentation performance on the GTA5-to-Cityscapes scenario, a widely used benchmark setting in unsupervised domain adaptation.
ICASSP.pdf
