Documents
Presentation Slides
Model Uncertainty for Unsupervised Domain Adaptation
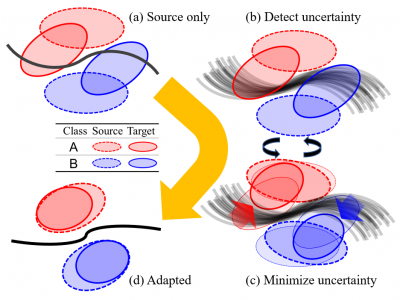
- Citation Author(s):
- Submitted by:
- JoonHo Lee
- Last updated:
- 3 November 2020 - 8:50am
- Document Type:
- Presentation Slides
- Document Year:
- 2020
- Event:
- Presenters:
- JoonHo Lee
- Paper Code:
- 2684
- Categories:
- Log in to post comments
The key principle of unsupervised domain adaptation is to minimize the divergence between source and target domain. Many recent methods follow this principle to learn domain-invariant features. They train task-specific classifiers to maximize the divergence and feature extractors to minimize the divergence in an adversarial way. However, this strategy often limits their performance. In this paper, we present a novel method that learns feature representations that minimize the domain divergence. We show that model uncertainty is a useful surrogate for the domain divergence. Our domain adaptation method based on model uncertainty (MUDA) employs Bayesian approach and provides an efficient way of evaluating model uncertainty loss using Monte Carlo dropout sampling. Experimental results on the image classification benchmarks show that our method is superior or comparable to state-of-the-art methods.