Documents
Presentation Slides
Sparsity preserved canonical correlation analysis
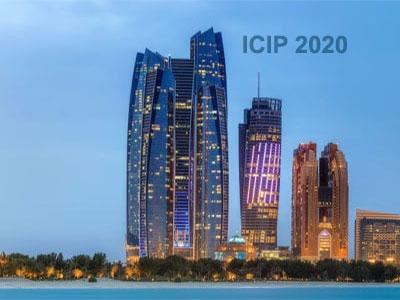
- Citation Author(s):
- Submitted by:
- Muhammad Qadar
- Last updated:
- 5 November 2020 - 3:01am
- Document Type:
- Presentation Slides
- Document Year:
- 2020
- Event:
- Presenters:
- Muhammad Ali Qadar
- Paper Code:
- 3200
- Categories:
- Log in to post comments
Canonical correlation analysis (CCA) describes the relationship between two sets of variables by finding linear combinations of the variables with maximal correlation. Recently, under the assumption that the leading canonical correlation directions are sparse, various procedures have been proposed for many high-dimensional applications to improve the interpretability of CCA. However all these procedures have the inconvenience of not preserving the sparsity among the retained leading canonical directions. To address this issue, a new sparse CCA method is proposed in this paper. It is derived within a penalized alternative least squares framework where the l2-norm is used as a penalty promoting block sparsity. The proposed method has the advantage of generating the same sparsity pattern across all retained canonical correlation components. The performance of the proposed method is demonstrated in a simulation study.