Documents
Poster
JOINT MASKED CPC AND CTC TRAINING FOR ASR
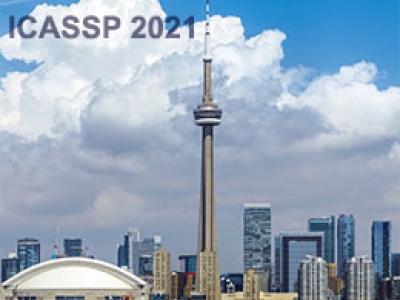
- Citation Author(s):
- Submitted by:
- Tatiana Likhomanenko
- Last updated:
- 2 September 2021 - 1:13am
- Document Type:
- Poster
- Document Year:
- 2021
- Event:
- Presenters:
- Tatiana Likhomanenko
- Paper Code:
- MLSP-11.5
- Categories:
- Log in to post comments
Self-supervised learning (SSL) has shown promise in learning representations of audio that are useful for automatic speech recognition (ASR). But, training SSL models like wav2vec~2.0 requires a two-stage pipeline. In this paper we demonstrate a single-stage training of ASR models that can utilize both unlabeled and labeled data. During training, we alternately minimize two losses: an unsupervised masked Contrastive Predictive Coding (CPC) loss and the supervised audio-to-text alignment loss Connectionist Temporal Classification (CTC). We show that this joint training method directly optimizes performance for the downstream ASR task using unsupervised data while achieving similar word error rates to wav2vec~2.0 on the Librispeech 100-hour dataset. Finally, we postulate that solving the contrastive task is a regularization for the supervised CTC loss.