Documents
Poster
IMAGE DEBLURRING BASED ON LIGHTWEIGHT MULTI-INFORMATION FUSION NETWORK
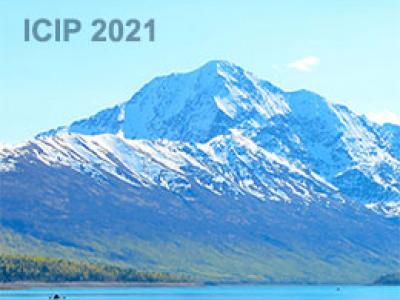
- Citation Author(s):
- Submitted by:
- yanni zhang
- Last updated:
- 23 September 2021 - 8:26pm
- Document Type:
- Poster
- Document Year:
- 2021
- Event:
- Presenters:
- Yanni Zhang
- Paper Code:
- ICIP-1701
- Categories:
- Log in to post comments
Recently, deep learning based image deblurring has been well
developed. However, exploiting the detailed image features in a
deep learning framework always requires a mass of parameters,
which inevitably makes the network suffer from high computational
burden. To solve this problem, we propose a lightweight multi-
information fusion network (LMFN) for image deblurring. The
proposed LMFN is designed as an encoder-decoder architecture. In
the encoding stage, the image feature is reduced to various small-
scale spaces for multi-scale information extraction and fusion
without a large amount of information loss. Then, a distillation
network is used in the decoding stage, which allows the network
benefit the most from residual learning while remaining sufficiently
lightweight. Meanwhile, an information fusion strategy between
distillation modules and feature channels is also carried out by
attention mechanism. Through fusing different information in the
proposed approach, our network can achieve state-of-the-art image
deblurring result with smaller number of parameters and
outperforms existing methods in model complexity.