Documents
Presentation Slides
Graph-based Transform based on 3D Convolutional Neural Network for Intra-Prediction of Imaging Data
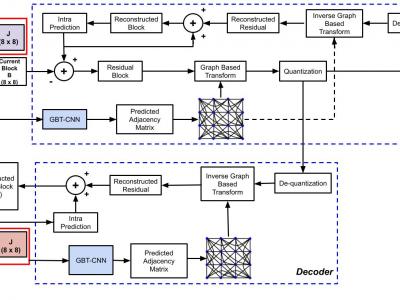
- Citation Author(s):
- Submitted by:
- Debaleena Roy
- Last updated:
- 5 March 2022 - 5:07pm
- Document Type:
- Presentation Slides
- Document Year:
- 2022
- Event:
- Presenters:
- Debaleena Roy
- Paper Code:
- DCC-197
- Categories:
- Log in to post comments
This paper presents a novel class of Graph-based Transform based on 3D convolutional neural networks (GBT-CNN) within the context of block-based predictive transform coding of imaging data. The proposed GBT-CNN uses a 3D convolutional neural network (3D-CNN) to predict the graph information needed to compute the transform and its inverse, thus reducing the signalling cost to reconstruct the data after transformation. The GBT-CNN outperforms the DCT and DCT/DST, which are commonly employed in current video codecs, in terms of the percentage of energy preserved by a subset of transform coefficients, the mean squared error of the reconstructed data, and the transform coding gain according to evaluations on several video frames and medical images.