Documents
Research Manuscript
EXPLORING EFFECTIVE DATA UTILIZATION FOR LOW-RESOURCE SPEECH RECOGNITION
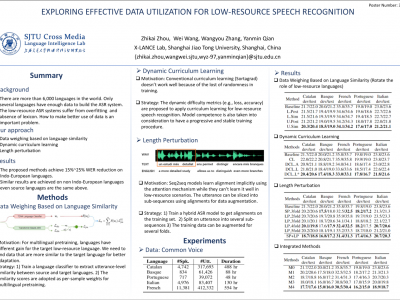
- Citation Author(s):
- Submitted by:
- Zhikai Zhou
- Last updated:
- 5 May 2022 - 5:01am
- Document Type:
- Research Manuscript
- Document Year:
- 2022
- Event:
- Presenters:
- Zhikai Zhou
- Paper Code:
- SPE-76.3
- Categories:
- Log in to post comments
Automatic speech recognition (ASR) has suffered great performance degradation when facing low-resource languages with limited training data. In this work, we propose a series of training strategies to explore more effective data utilization for low-resource speech recognition. In low-resource scenarios, multilingual pretraining is of great help for the above purpose. We exploit relationships among different languages for better pretraining. Then, the knowledge extracted from the language classifier is utilized for data weighing on training samples, making the model more biased towards the target low-resource language. Moreover, dynamic curriculum learning as a warm-up strategy and length perturbation as data augmentation are also designed. All these three methods form a newly improved training strategy for low-resource speech recognition. Meanwhile, we evaluate the proposed strategies using rich-resource languages for pretraining (PT) and finetuning (FT) the model on the target language with limited data. The experimental results show that on the CommonVoice dataset, compared with the commonly used multilingual PT+FT method, the proposed strategies achieve a relative 15-25% reduction in word error rate on different target languages, which shows the significant effects of the proposed data utilization strategy.