Documents
Presentation Slides
DENOISING-GUIDED DEEP REINFORCEMENT LEARNING FOR SOCIAL RECOMMENDATION
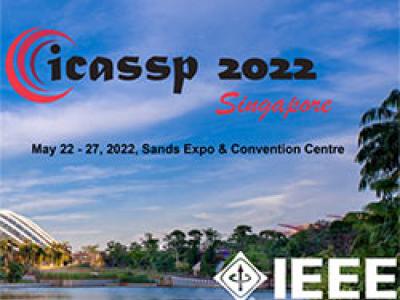
- Citation Author(s):
- Submitted by:
- qihan Du
- Last updated:
- 10 May 2022 - 1:40am
- Document Type:
- Presentation Slides
- Document Year:
- 2022
- Event:
- Presenters:
- Qihan Du
- Paper Code:
- MLSP-35.5
- Categories:
- Keywords:
- Log in to post comments
Social recommendation (SR) aims to enhance the performance of recommendations by incorporating social information. However, such information is not always reliable, e.g., some of the friends may share similar preferences with the user on a specific item, while others may be irrelevant to this item due to domain differences. Therefore, modeling all of the user's social relationships without considering the relevance of friends will introduce noises to the social context. To address this issue, in this work, we propose a Denoising-guided deep Reinforcement Learning framework for Social recommendation (DRL4So). Specifically, the agent (i.e., social denoiser) in our framework automatically masks the user's friends who are irrelevant to the target item; Then, the environment (i.e., recommender) is designed to give rewards to the agent for social denoising without supervised signals; Finally, the two components are jointly trained by DPG to ensure that social denoising correctly guides the recommendation. We conduct extensive experiments on three public datasets, and the results show that DRL4So outperforms existing state-of-the-art SR methods (improving 38.67% and 19.81% in terms of HR@10 and NDCG@10, respectively).
DRL4So.pptx
