Documents
Poster
Group nonnegative matrix factorisation with speaker and session variability compensation for speaker identification
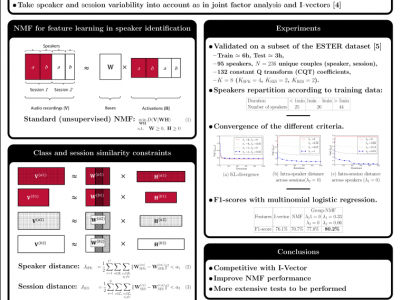
- Citation Author(s):
- Submitted by:
- romain serizel
- Last updated:
- 14 March 2016 - 6:42am
- Document Type:
- Poster
- Document Year:
- 2016
- Event:
- Presenters:
- Romain Serizel
- Categories:
- Log in to post comments
This paper presents a feature learning approach for speaker identification that is based on nonnegative matrix factorisation. Recent studies have shown that with such models, the dictionary atoms can represent well the speaker identity. The approaches proposed so far focused only on speaker variability and not on session variability. However, this later point is a crucial aspect in the success of the I-vector approach that is now the state-of-the-art in speaker identification.
This paper proposes a method that relies on group nonnegative matrix factorisation and that is inspired by the I-vector training procedure. By doing so the proposed approach intends to capture both the speaker variability and the session variability. Results on a small corpus prove that the proposed approach can be competitive with I-vectors.