Documents
Poster
Evaluating Speech–Phoneme Alignment and Its Impact on Neural Text-To-Speech Synthesis
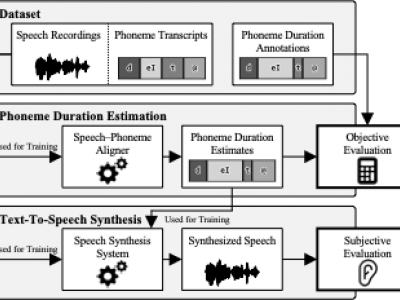
- Citation Author(s):
- Submitted by:
- Frank Zalkow
- Last updated:
- 30 May 2023 - 2:09am
- Document Type:
- Poster
- Document Year:
- 2023
- Event:
- Presenters:
- Frank Zalkow
- Paper Code:
- 663
- Categories:
- Log in to post comments
In recent years, the quality of text-to-speech (TTS) synthesis vastly improved due to deep-learning techniques, with parallel architectures, in particular, providing excellent synthesis quality at fast inference. Training these models usually requires speech recordings, corresponding phoneme-level transcripts, and the temporal alignment of each phoneme to the utterances. Since manually creating such fine-grained alignments requires expert knowledge and is time-consuming, it is common practice to estimate them using automatic speech–phoneme alignment methods. In the literature, either the estimation methods' accuracy or their impact on the TTS system's synthesis quality is evaluated. In this study, we perform experiments with five state-of-the-art speech–phoneme aligners and evaluate their output with objective and subjective measures. As our main result, we show that small alignment errors (below 75 ms error) do not decrease the synthesis quality, which implies that the alignment error may not be the crucial factor when choosing an aligner for TTS training.