Documents
Presentation Slides
Feature integration via back-projection orderingmulti-modal Gaussian process latent variable modelfor rating prediction
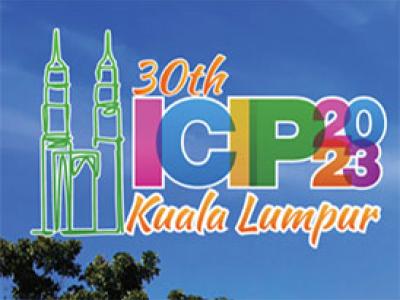
- DOI:
- 10.60864/y71z-tm97
- Citation Author(s):
- Submitted by:
- Kyohei Kamikawa
- Last updated:
- 17 November 2023 - 12:05pm
- Document Type:
- Presentation Slides
- Document Year:
- 2023
- Event:
- Presenters:
- Kyohei Kamikawa
- Paper Code:
- 3047
- Categories:
- Log in to post comments
In this paper, we present a method of feature integration via backprojection ordering multi-modal Gaussian process latent variable model (BPomGP) for rating prediction. In the proposed method, to extract features reflecting the users’ interest, we use the known ratings assigned to the viewed contents and users’ behavior information while viewing the contents which is related to the users’ interest. BPomGP has two important approaches. Unlike the training phase, where the above two types of heterogeneous information are available, behavior information is not given in the test phase. Thus, the projection that transforms content features into integrated features is trained by using both content and behavior features. Furthermore, to reflect the unique characteristics of ratings, their ordering, we constrain the distance between integrated features in a latent space based on the difference in the degree of known ratings. Finally, experimental results show that the proposed method can calculate features that are useful for rating prediction.