Documents
Research Manuscript
SegGuard: Defending Scene Segmentation against Adversarial Patch Attack - Supplementary Material
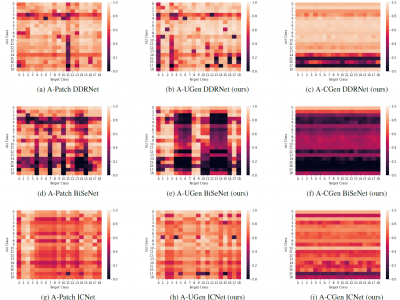
- Citation Author(s):
- Submitted by:
- John Collomosse
- Last updated:
- 31 January 2024 - 6:51am
- Document Type:
- Research Manuscript
- Document Year:
- 2024
- Event:
- Presenters:
- John Collomosse
- Paper Code:
- 1676
- Categories:
- Log in to post comments
Adversarial Patch Attacks (APAs) induce prediction errors by inserting carefully crafted regions into images. This paper presents the first defence against APAs for deep networks that perform semantic segmentation of scenes. We show that a conditional generator can be trained to produce patches on demand targeting specific classes and achieving superior performance versus conventional pixel-optimised patch attacks. We then leverage this generator along with the segmentation network as part of a generative adversarial network, which trains the model to ignore the adversarial patches produced by the generator, while simultaneously training the generator to produce updated patches to attack the fine-tuned network. We show that our process confers strong protection against adversarial patches, and that this protection generalises to traditional pixel-optimised adversarial patches.