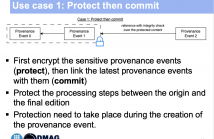
The surge of media consumption on the Internet reflects how individuals engage with information, entertainment and communication. In parallel, the advancement of generative AI tools facilitates the creation of abundant content that is nearly indistinguishable from authentic content. To address aspects of misinformation, focus is shifted towards the secure and immutable annotation of provenance information. Although such frameworks aim to establish trust in media consumption, they raise privacy concerns, as provenance data may conceal identifiable information for individuals and locations.
- Categories:
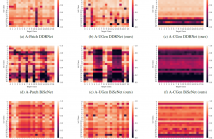
- Read more about SegGuard: Defending Scene Segmentation against Adversarial Patch Attack - Supplementary Material
- Log in to post comments
Adversarial Patch Attacks (APAs) induce prediction errors by inserting carefully crafted regions into images. This paper presents the first defence against APAs for deep networks that perform semantic segmentation of scenes. We show that a conditional generator can be trained to produce patches on demand targeting specific classes and achieving superior performance versus conventional pixel-optimised patch attacks.
- Categories:
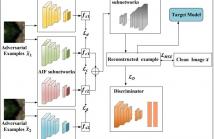
- Read more about Adversarial Defense via Perturbation-Disentanglement in Hyperspectral Image Classification
- Log in to post comments
Template.pdf

- Categories:
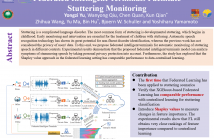
- Read more about Federated Intelligent Terminals Facilitate Stuttering Monitoring
- Log in to post comments
Stuttering is a complicated language disorder. The most common form of stuttering is developmental stuttering, which begins in childhood. Early monitoring and intervention are essential for the treatment of children with stuttering. Automatic speech recognition technology has shown its great potential for non-fluent disorder identification, whereas the previous work has not considered the privacy of users' data. To this end, we propose federated intelligent terminals for automatic monitoring of stuttering speech in different contexts.
- Categories:
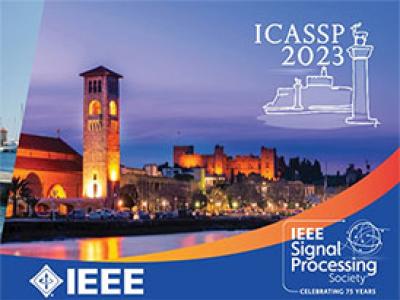
- Read more about InfoShape: Task-Based Neural Data Shaping via Mutual Information
- Log in to post comments
The use of mutual information as a tool in private data sharing has remained an open challenge due to the difficulty of its estimation in practice. In this paper, we propose InfoShape, a task-based encoder that aims to remove unnecessary sensitive information from training data while maintaining enough relevant information for a particular ML training task. We achieve this goal by utilizing mutual information estimators that are based on neural networks, in order to measure two performance metrics, privacy and utility.
- Categories:
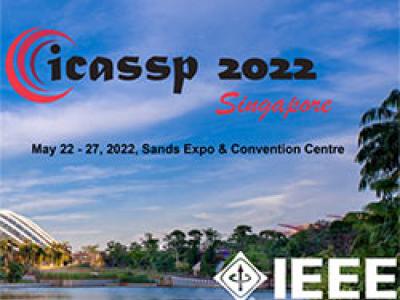
- Read more about Compressed Data Sharing based on Information Bottleneck Model
- Log in to post comments
In this paper, we consider privacy-preserving compressed image sharing, where the goal is to release compressed data whilst satisfying some privacy/secrecy constraints yet ensuring image reconstruction with a defined fidelity. The privacy-preserving compressed image sharing is addressed using a machine learning framework based on an information bottleneck with a shared secret key for authorized users. In contrast, an adversary observing the protected compressed representation tries to either reconstruct the data or deduce some privacy-sensitive attributes such as gender, age, etc.
- Categories:
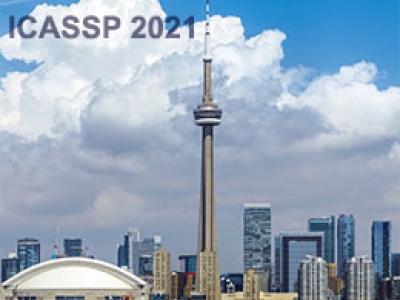
- Read more about ATTACK ON PRACTICAL SPEAKER VERIFICATION SYSTEM USING UNIVERSAL ADVERSARIAL PERTURBATIONS
- Log in to post comments
5375slide.pdf

- Categories:
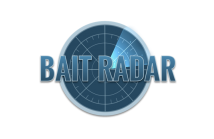
- Read more about BAITRADAR: A MULTI-MODEL CLICKBAIT DETECTION ALGORITHM USING DEEP LEARNING
- Log in to post comments
- Categories:
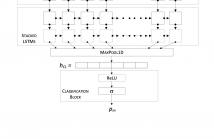
- Read more about Detection of Malicious VBScript Using Static and Dynamic Analysis with Recurrent Deep Learning
- Log in to post comments
- Categories:
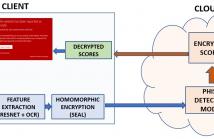
- Read more about Privacy-Preserving Phishing Web Page Classification Via Fully Homomorphic Encryption
- Log in to post comments
- Categories: