Documents
Supplementary Materials
Supplementary Materials: Self-supervised disentangled representation learning of artistic style through Neural Style Transfer
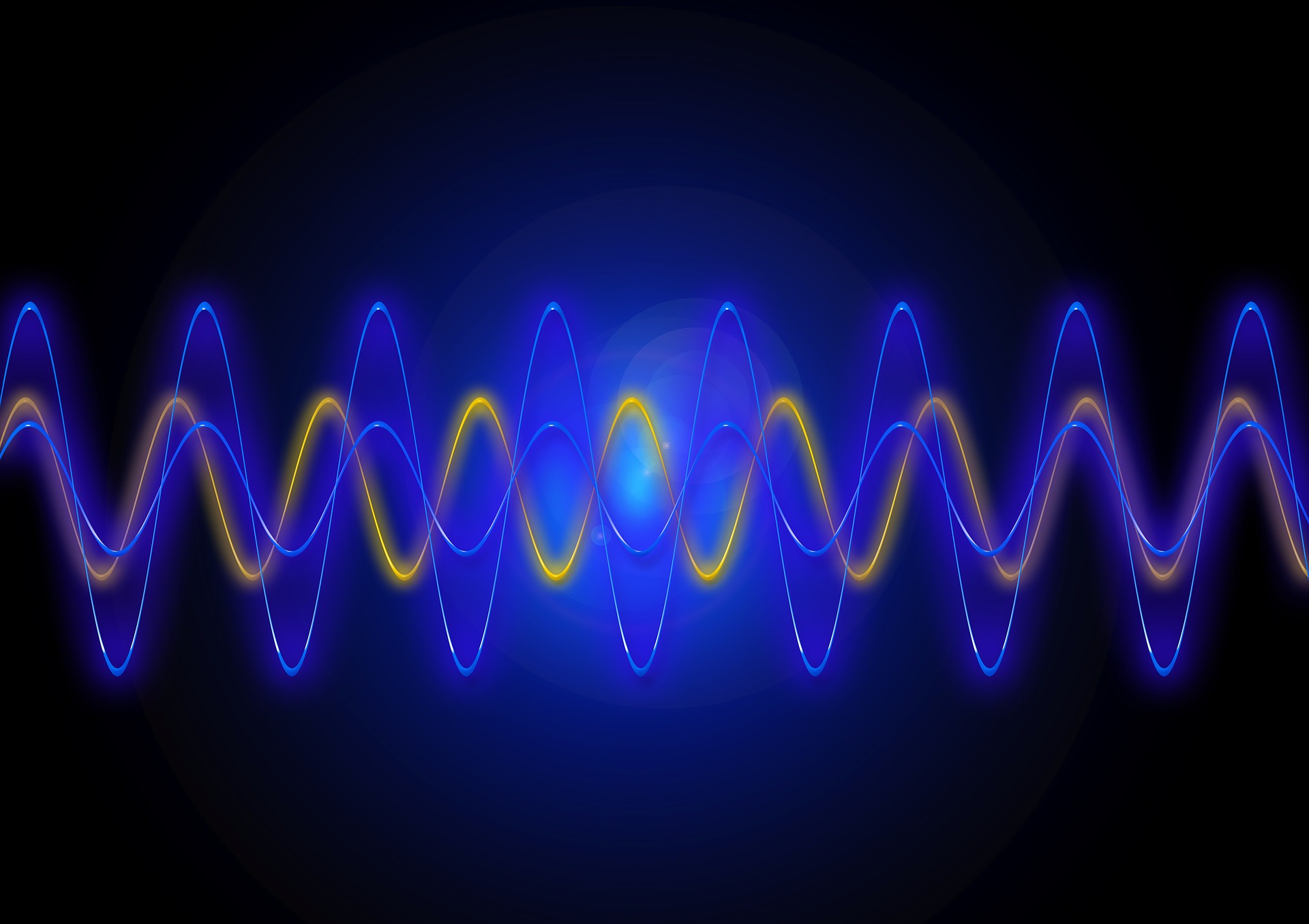
- Citation Author(s):
- Submitted by:
- Dan Ruta
- Last updated:
- 31 January 2024 - 3:32pm
- Document Type:
- Supplementary Materials
- Document Year:
- 2024
- Categories:
- Log in to post comments
We present a new method for learning a fine-grained representation of visual style. Representation learning aims to discover individual salient features of a domain in a compact and descriptive form that strongly identifies the unique characteristics of that domain. Prior visual style representation works attempt to disentangle style (ie appearance) from content (ie semantics) yet a complete separation has yet to be achieved. We present a technique to learn a representation of visual style more strongly disentangled from the semantic content depicted in an image. We use Neural Style Transfer (NST) to measure and drive the learning signal and achieve state-of-the-art representation learning on explicitly disentangled metrics. We show that strongly addressing the disentanglement of style and content leads to large gains in style-specific metrics, encoding far less semantic information and achieving state-of-the-art accuracy in downstream style matching (retrieval) and zero-shot style tagging tasks.