Documents
Presentation Slides
Video-Language Graph Convolutional Network for Human Action Recognition
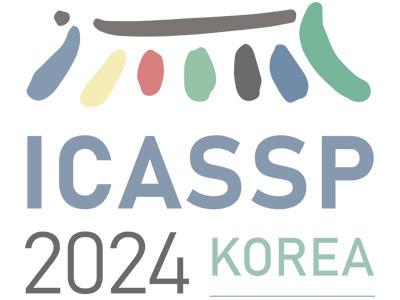
- DOI:
- 10.60864/3ztw-x820
- Citation Author(s):
- Submitted by:
- Rui Zhang
- Last updated:
- 6 June 2024 - 10:32am
- Document Type:
- Presentation Slides
- Document Year:
- 2024
- Event:
- Presenters:
- Rui Zhang
- Paper Code:
- MMSP-L4.4
- Categories:
- Log in to post comments
Transferring visual language models (VLMs) from the image domain to the video domain has recently yielded great success on human action recognition tasks. However, standard recognition paradigms overlook fine-grained action parsing knowledge that could enhance the recognition accuracy. In this paper, we propose a novel method that leverages both coarse-grained and fine-grained knowledge to recognize human actions in videos. Our method consists of a video-language graph convolutional network that integrates and fuses multi-modal knowledge in a progressive manner. We evaluate our method on the Kinetics-TPS, a large-scale action parsing dataset, and demonstrate that it outperforms the state-of-the-art methods by a significant margin. Moreover, our method achieves better results with less training data and competitive computational cost than the existing methods, showing the effectiveness and efficiency of using fine-grained knowledge for human video action recognition.