Documents
Poster
DOMAIN-WISE INVARIANT LEARNING FOR PANOPTIC SCENE GRAPH GENERATION
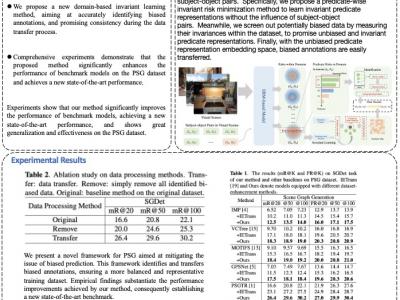
- Citation Author(s):
- Submitted by:
- Li Li
- Last updated:
- 1 April 2024 - 2:23am
- Document Type:
- Poster
- Document Year:
- 2024
- Event:
- Presenters:
- Li Li
- Paper Code:
- IVMSP-P10.3
- Categories:
- Log in to post comments
Panoptic Scene Graph Generation (PSG) involves the detection of objects and the prediction of their corresponding relationships (predicates). However, the presence of biased predicate annotations poses a significant challenge for PSG models, as it hinders their ability to establish a clear decision boundary among different predicates. This issue substantially impedes the practical utility and real-world applicability of PSG models.
To address the intrinsic bias above, we propose a novel framework to infer potentially biased annotations by measuring the predicate prediction risks within each subject-object pair (domain), and adaptively transfer the biased annotations to consistent ones by learning invariant predicate representation embeddings.
Experiments show that our method significantly improves the performance of benchmark models, achieving a new state-of-the-art performance, and shows great generalization and effectiveness on PSG dataset.