Documents
Presentation Slides
META REPRESENTATION LEARNING METHOD FOR ROBUST SPEAKER VERIFICATION IN UNSEEN DOMAINS
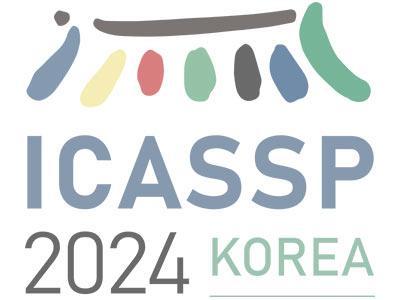
- Citation Author(s):
- Submitted by:
- Jian-Tao Zhang
- Last updated:
- 1 April 2024 - 2:01pm
- Document Type:
- Presentation Slides
- Document Year:
- 2024
- Event:
- Presenters:
- Jian-Tao Zhang
- Paper Code:
- SLP-L9.3
- Categories:
- Log in to post comments
This paper presents a meta representation learning method for robust speaker verification (SV) in unseen domains. It is known that the existing embedding learning based SV systems may suffer from domain mismatch issues. To address this, we propose an episodic training procedure to compensate domain mismatch conditions at runtime. Specifically, episodes are constructed with domain balanced episodic sampling from two different domains, and a new domain alignment (DA) module is added besides the feature extractor (FE) and classifier to existing network structures. In each episodic training iteration, FE and DA modules are optimized separately with different objectives to improve the robustness of learning. Besides, a cross-domain inter-class alignment (CDICA) loss is proposed for improving the domain generalization ability. Experimental results on CNCeleb and VoxCeleb benchmarks demonstrate significant performance gains for unseen domains in SV.