Documents
Poster
PRE-TRAINED ACOUSTIC-AND-TEXTUAL MODELING FOR END-TO-END SPEECH-TO-TEXT TRANSLATION
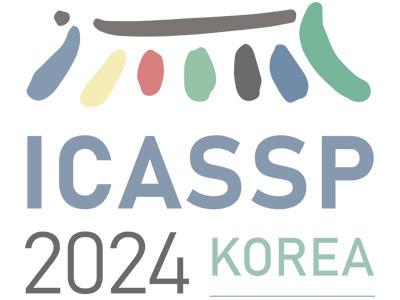
- DOI:
- 10.60864/8bcm-t971
- Citation Author(s):
- Submitted by:
- Weitai Zhang
- Last updated:
- 2 April 2024 - 2:59am
- Document Type:
- Poster
- Document Year:
- 2024
- Event:
- Presenters:
- Zhongyi Ye
- Paper Code:
- SLP-P23.3
- Categories:
- Log in to post comments
End-to-end paradigm has aroused more and more interests and attention for improving speech-to-text translation (ST) recently. Existing end-to-end models mainly attributes and attempts to address the problem of modeling burden and data scarcity, while always fail to maintain both cross-modal and cross-lingual mapping well at the same time.
In this work, we investigate methods for improving end-to-end ST with pre-trained acoustic-and-textual models. Our acoustic encoder and decoder begins with processing the source speech sequence as usual. A textual encoder and an adaptor module then obtain source acoustic and textual information respectively, alleviating the representation inconsistency with attentive interactions in the textual decoder. Also, we utilize pre-trained models, and develop an adaptation fine-tuning method to preserve the pre-training knowledge.
Experimental results on the IWSLT2023 offline ST task from English to German, Japanese and Chinese show that our method achieves state-of-the-art BLEU scores and surpasses the strong cascaded ST counterparts in unrestricted setting.