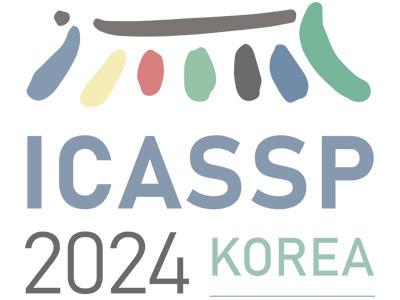
- Read more about PRE-TRAINED ACOUSTIC-AND-TEXTUAL MODELING FOR END-TO-END SPEECH-TO-TEXT TRANSLATION
- Log in to post comments
End-to-end paradigm has aroused more and more interests and attention for improving speech-to-text translation (ST) recently. Existing end-to-end models mainly attributes and attempts to address the problem of modeling burden and data scarcity, while always fail to maintain both cross-modal and cross-lingual mapping well at the same time.
- Categories:
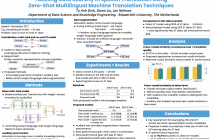
- Read more about Tackling Data Scarcity in Speech Translation Using Zero-Shot Multilingual Machine Translation Techniques
- Log in to post comments
Recently, end-to-end speech translation (ST) has gained significant attention as it avoids error propagation. However, the approach suffers from data scarcity. It heavily depends on direct ST data and is less efficient in making use of speech transcription and text translation data, which is often more easily available. In the related field of multilingual text translation, several techniques have been proposed for zero-shot translation. A main idea is to increase the similarity of semantically similar sentences in different languages.
- Categories:
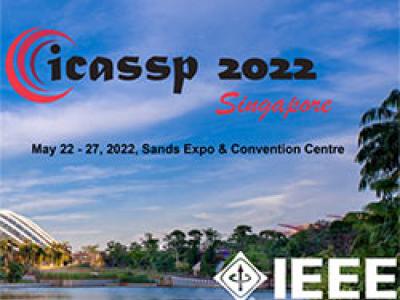
- Read more about Integrating multiple ASR systems into NLP backend with attention fusion
- Log in to post comments
- Categories:
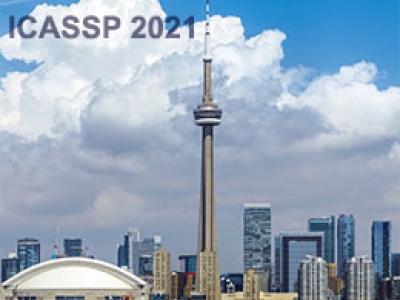
- Read more about MODELING HOMOPHONE NOISE FOR ROBUST NEURAL MACHINE TRANSLATION
- Log in to post comments
- Categories:
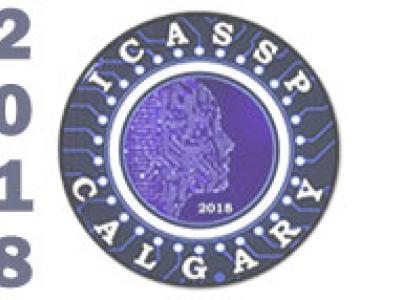
- Read more about ANALYSIS OF MULTILINGUAL BLSTM ACOUSTIC MODEL ON AND HIGH RESOURCE LANGUAGES
- Log in to post comments
The paper provides an analysis of automatic speech recognition
systems (ASR) based on multilingual BLSTM, where we used multi-task
training with separate classification layer for each language. The
focus is on low resource languages, where only a limited
amount of transcribed speech is available. In such
scenario, we found it
essential to train the ASR systems in a multilingual fashion and we
report superior results
obtained with pre-trained multilingual BLSTM on this task.
The high resource languages are also
poster.pdf

- Categories:
- Read more about Prosodic Annotation Enriched Statistical Machine Translation
- Log in to post comments
More and more linguistic information has been employed to improve the performance of machine translation, such as part of speech, syntactic structures, discourse contexts, and so on. However, conventional approaches typically ignore the key information beyond the text such as prosody. In this paper, we exploit and employ three prosodic features: pronunciation (phonetic alphabet and tone), prosodic boundaries and emphasis.
- Categories: