Documents
Poster
Poster for "Graph-based permutation patterns for the analysis of task-related fMRI signals on DTI networks in mild cognitive impairment"
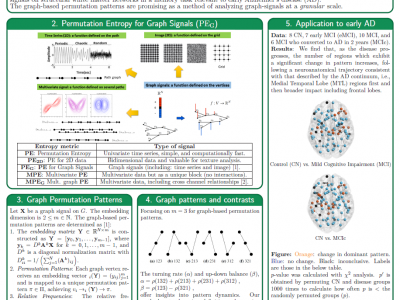
- Citation Author(s):
- Submitted by:
- Javier Escudero
- Last updated:
- 8 April 2024 - 5:45pm
- Document Type:
- Poster
- Document Year:
- 2024
- Event:
- Presenters:
- Javier Escudero
- Paper Code:
- BISP-P9.2
- Categories:
- Keywords:
- Log in to post comments
Permutation Entropy (PE) is a powerful nonlinear analysis technique for univariate time series. Recently, Permutation Entropy for Graph signals (PE_G) has been proposed to extend PE to data residing on irregular domains. However, PE_G is limited as it provides a single value to characterise a whole graph signal. Here, we introduce a novel approach to evaluate graph signals at the vertex level: graph-based permutation patterns. Synthetic datasets show the efficacy of our method. We reveal that dynamics in graph signals, undetectable with PE_G , can be discerned using our graph-based patterns. These are then validated in DTI and fMRI data acquired during a working memory task in mild cognitive impairment, where we explore functional brain signals on structural white matter networks. Our findings suggest that graph-based permutation patterns in individual brain regions change as the disease progresses, demonstrating potential as a method of analyzing graph-signals at a granular scale.