Documents
Presentation Slides
GLAND SEGMENTATION VIA DUAL ENCODERS AND BOUNDARY-ENHANCED ATTENTION
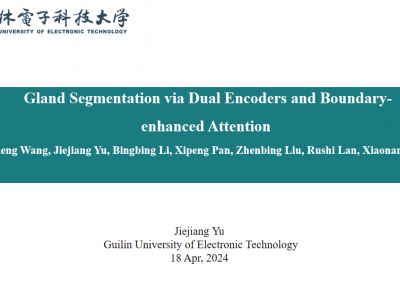
- DOI:
- 10.60864/0tpf-ak87
- Citation Author(s):
- Submitted by:
- jiejiang Yu
- Last updated:
- 6 June 2024 - 10:28am
- Document Type:
- Presentation Slides
- Document Year:
- 2024
- Event:
- Presenters:
- Jiejiang Yu
- Paper Code:
- BISP-L5.6
- Categories:
- Keywords:
- Log in to post comments
Accurate and automated gland segmentation on pathological images can assist pathologists in diagnosing the malignancy of colorectal adenocarcinoma. However, due to various gland shapes, severe deformation of malignant glands, and overlapping adhesions between glands. Gland segmentation has always been very challenging. To address these problems, we propose a DEA model. This model consists of two branches: the backbone encoding and decoding network and the local semantic extraction network. The backbone encoding and decoding network extracts advanced Semantic features, uses the proposed feature decoder to restore feature space information, and then enhances the boundary features of the gland through boundary enhancement attention. The local semantic extraction network uses the pre-trained DeepLabv3+ as a Local semantic-guided encoder to realize the extraction of edge features. Experimental results on two public datasets, GlaS and CRAG, confirm that the performance of our method is better than other gland segmentation methods.