Documents
Poster
Image Mixing and Gradient Smoothing to Enhance the SAR Image Attack
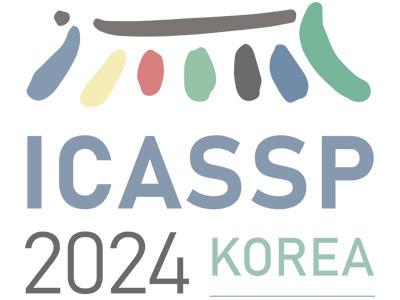
- DOI:
- 10.60864/95wq-zv29
- Citation Author(s):
- Submitted by:
- Xin Liu
- Last updated:
- 6 June 2024 - 10:50am
- Document Type:
- Poster
- Document Year:
- 2024
- Event:
- Presenters:
- Xin Liu
- Paper Code:
- 2343
- Categories:
- Log in to post comments
Deep Neural Networks (DNNs) are known to be vulnerable to adversarial examples, which are crafted by adding imperceptible perturbations to clean examples. With the wide applications of DNNs to Synthetic Aperture Radar (SAR) Automatic Target Recognition (ATR), the vulnerability of SAR deep recognition models has attracted increasing attention. Existing works show that input transformation can effectively improve the black-box attack performance of adversarial examples, but there is little work in the field of SAR-ATR.In this paper, we propose a novel input transformation attack called Image Mixing and Gradient Smoothing (IMGS), which is dedicated to attacking SAR images. Considering that SAR images are composed of amplitude and phase components, IMGS mixes a small portion of another image into the input samples in amplitude and phase with different mixing rates, respectively. Extensive experiments conducted on the MSTAR dataset demonstrate that IMGS significantly outperforms other input transformation methods, originally designed for attacking visual images, in both white-box and black-box settings.