Documents
Poster
Real-Time Privacy-Preserving Fall Risk Assessment with a Single Body-Worn Tracking Camera
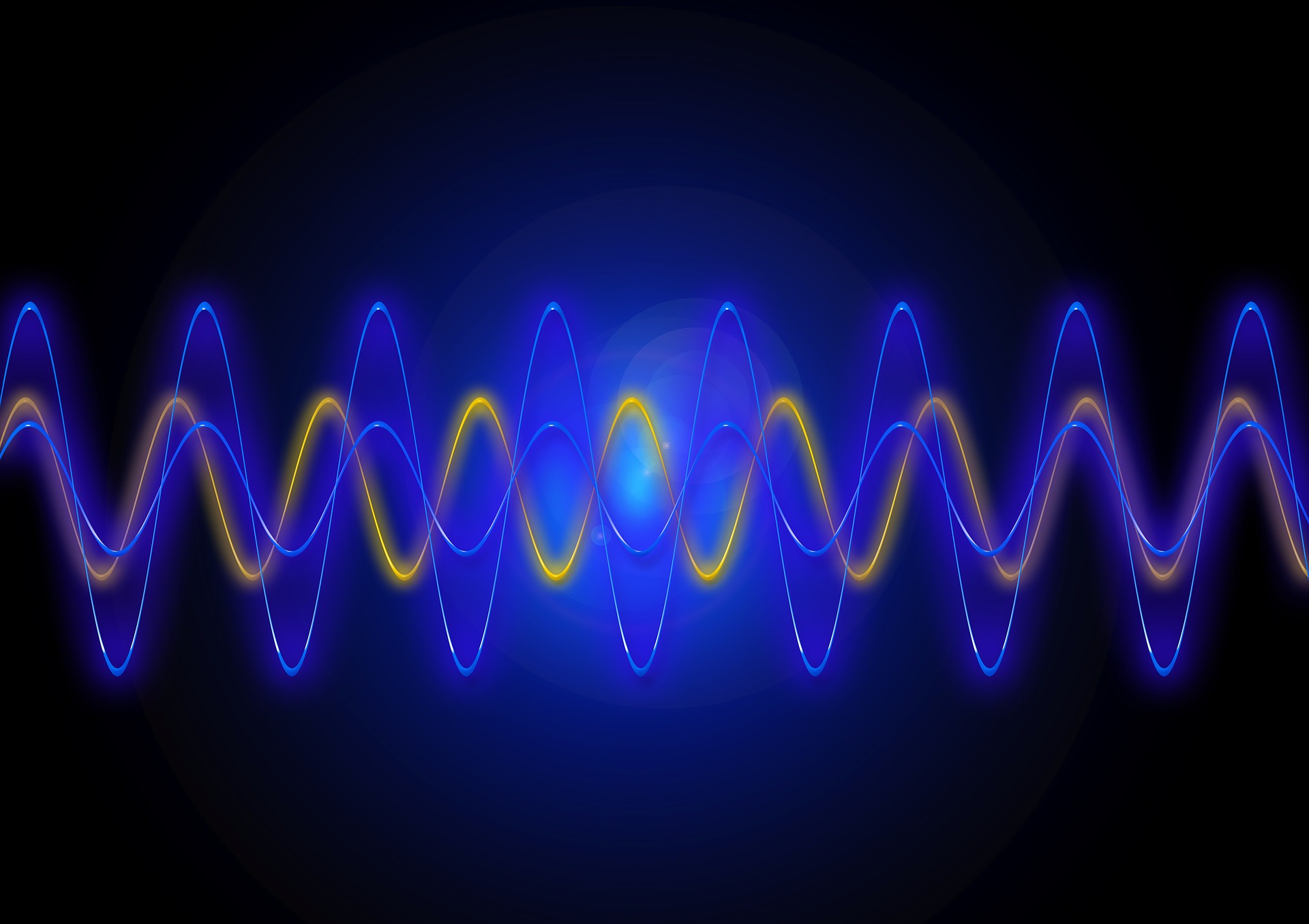
- DOI:
- 10.60864/6q8f-sr03
- Citation Author(s):
- Submitted by:
- Chiao-Yi Wang
- Last updated:
- 6 June 2024 - 10:27am
- Document Type:
- Poster
- Categories:
- Log in to post comments
Falls are a major cause of injury among elderly adults. In addition, recent research indicates that elderly adults often fall because of the same individual biomechanical causes. Therefore, real-time individual fall risk assessment is crucial for designing a more effective and personalized fall reduction training program. However, existing methods for fall risk assessment either raise privacy concerns or require multiple wearable sensors. In this paper, we propose EgoFall, a real-time privacy-preserving fall risk assessment system using a commercial tracking camera. EgoFall utilizes a chest-mounted tracking camera and a carefully designed data pre-processing pipeline to acquire the ego-body motion data of the subject. The ego-body motion data is then fed to a lightweight CNNTransformer model for fall risk assessment. We demonstrate that EgoFall not only outperforms the baseline methods but also has very low computational complexity, which is highly suitable for real-time processing.