Documents
Presentation Slides
Personalised Anomaly Detectors and Prototypical Representations for Relapse Detection from Wearable-Based Digital Phenotyping
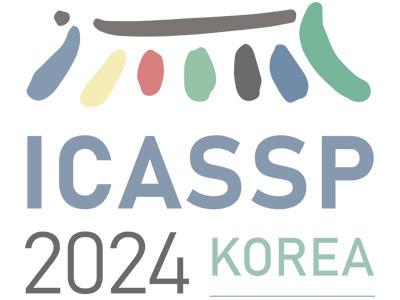
- Citation Author(s):
- Submitted by:
- Adria Mallol-Ragolta
- Last updated:
- 15 April 2024 - 9:49am
- Document Type:
- Presentation Slides
- Document Year:
- 2024
- Event:
- Presenters:
- Adria Mallol-Ragolta
- Paper Code:
- GC-L2.4
- Categories:
- Log in to post comments
We describe our contribution to the 2nd e-Prevention challenge, which focuses on the unsupervised non-psychotic (Track 1) and psychotic (Track 2) relapse detection using wearable-based digital phenotyping. We exploit the measurements gathered from the gyroscope, the accelerometer, and the heart rate-related sensors embedded in a smartwatch. We also include the available sleep information in our experiments. Four dedicated autoencoders are trained to learn embedded representations from each one of the considered modalities. The learnt embeddings are then used to compute personalised, non-relapse Elliptic Envelope anomaly detectors and prototypical representations of each patient. The Mahalanobis distance between the embeddings the autoencoders extract from unseen data and the training, non-relapse distribution determines the likelihood that the former corresponds to a relapse state. Our best systems achieve a macro-averaged AUROC and AUPRC score of 56.7 % and 49.9 % on the test sets of Track 1 and Track 2, respectively.