Documents
Poster
Privacy Preserving Federated Learning from Multi-input Functional Proxy Re-encryption
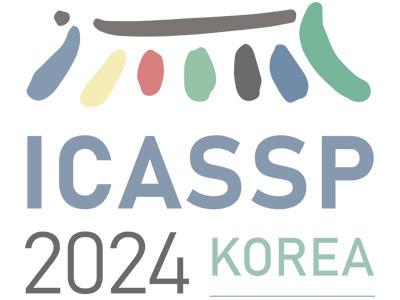
- Citation Author(s):
- Submitted by:
- Xinyu Feng
- Last updated:
- 15 April 2024 - 10:21am
- Document Type:
- Poster
- Document Year:
- 2024
- Event:
- Presenters:
- Xinyu Feng
- Paper Code:
- MLSP-P33.6
- Categories:
- Log in to post comments
Federated learning (FL) allows different participants to collaborate on model training without transmitting raw data, thereby protecting user data privacy. However, FL faces a series of security and privacy issues (e.g. the leakage of raw data from publicly shared parameters). Several privacy protection technologies, such as homomorphic encryption, differential privacy and functional encryption, are introduced for privacy enhancement in FL. Among them, the FL frameworks based on functional encryption better balance security and performance, thus receiving increasing attention. The previous FL frameworks based on functional encryption suffer from several security issues, including attacks by combining multiple rounds of ciphertexts and keys, and leakage of global parameters to the central server. To tackle these issues, we propose a novel multi-input functional proxy re-encryption (MI-FPRE) scheme and further design a new FL framework with better privacy based on MI-FPRE. Our framework allows a semi-trusted central server to aggregate the parameters without knowing the intermediate parameters and the result of aggregation, thus achieves better privacy in FL training. The experimental results indicate that our framework achieves less communication overhead and higher computational efficiency without losing accuracy.