Documents
Presentation Slides
slides for av2wav
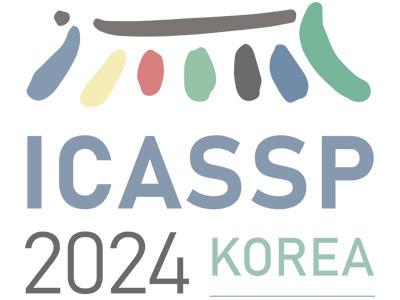
- DOI:
- 10.60864/e7pf-jn30
- Citation Author(s):
- Submitted by:
- Ju-Chieh Chou
- Last updated:
- 15 April 2024 - 10:17pm
- Document Type:
- Presentation Slides
- Document Year:
- 2024
- Event:
- Presenters:
- Ju-Chieh Chou
- Paper Code:
- SLP-L1.5
- Categories:
- Log in to post comments
Speech enhancement systems are typically trained using pairs of clean and noisy speech. In audio-visual speech enhancement
(AVSE), there is not as much ground-truth clean data available; most audio-visual datasets are collected in real-world environments with background noise and reverberation, hampering the development of AVSE. In this work, we introduce AV2Wav, a resynthesis-based audio-visual speech enhancement approach that can generate clean speech despite the challenges of real-world training data. We obtain a subset of nearly clean speech from an audio-visual corpus using a neural quality estimator, and then train a diffusion model on this subset to generate waveforms conditioned on continuous speech representations from AV-HuBERT with noise-robust training. We use continuous rather than discrete representations to retain prosody and speaker information. With this vocoding task alone, the model can perform speech enhancement better than a masking-based baseline. We further fine-tune the model on clean/noisy utterance pairs to improve the performance. Our approach outperforms a masking-based baseline in terms of both automatic metrics and a human listening test and is close in quality to the target speech in the listening test.
av2wav_pp.pptx

Comments
no
no