Documents
Poster
DISTRIBUTED STOCHASTIC CONTEXTUAL BANDITS FOR PROTEIN DRUG INTERACTION
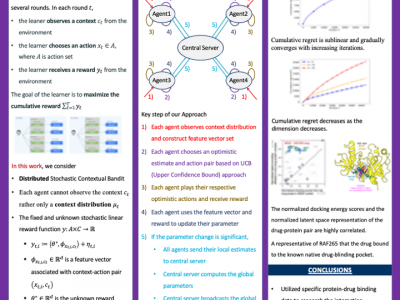
- Citation Author(s):
- Submitted by:
- Shana Moothedath
- Last updated:
- 15 April 2024 - 10:27pm
- Document Type:
- Poster
- Document Year:
- 2024
- Event:
- Presenters:
- Jiabin Lin and Ratul Chowdhury
- Paper Code:
- MLSP-P10.9
- Categories:
- Log in to post comments
In recent work [1], we developed a distributed stochastic multi-arm contextual bandit algorithm to learn optimal actions when the contexts are unknown, and M agents work collaboratively under the coordination of a central server to minimize the total regret. In our model, the agents observe only the context distribution and the exact context is unknown to the agents. Such a situation arises, for instance, when the context itself is a noisy measurement or based on a prediction mechanism. By performing a feature vector transformation and by leveraging the UCB algorithm, we proposed a UCB algorithm for stochastic bandits with context distribution. In this paper, we test our algorithm on a real-world dataset and investigate the interactions between drugs and proteins. For this, we perform a data pre-processing step to fit the model and we evaluated the performance of our algorithm for the drug-protein interaction study as compared to other benchmark algorithms. Furthermore, we present the results of biological experiments and draw inferences from our findings.
Comments
Thanks
Thanks