Documents
Supplementary material
Supplementary material for "Geometry Regularized Point Cloud Autoencoder"
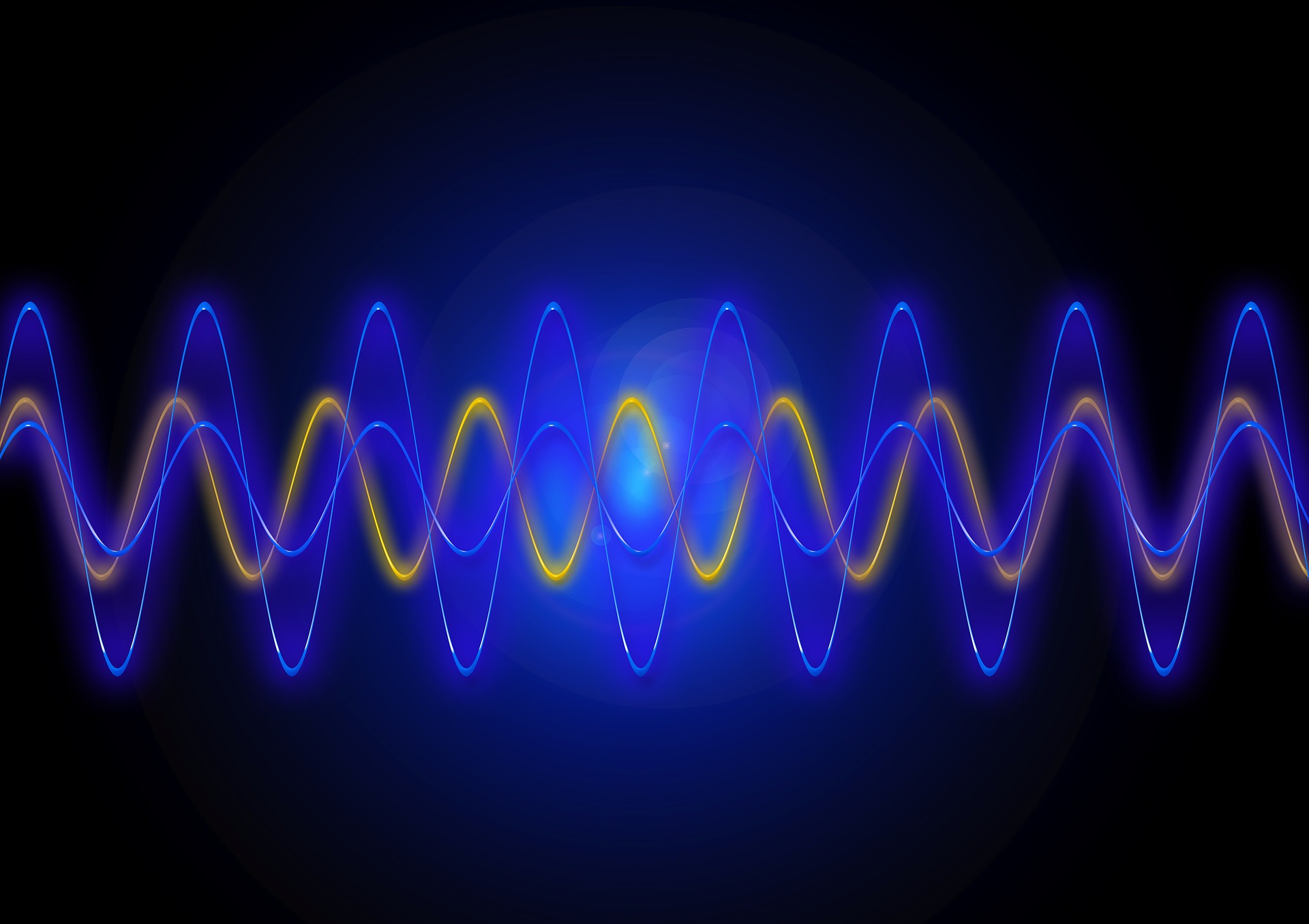
- DOI:
- 10.60864/8jjz-rs86
- Citation Author(s):
- Submitted by:
- Anonymous Author(s)
- Last updated:
- 21 January 2025 - 12:09pm
- Document Type:
- Supplementary material
- Categories:
- Log in to post comments
Point cloud is a prevalent format in representing 3D geometry. Regardless of the recent advances, unsupervised learning for 3D point clouds remains arduous for various tasks due to its unorganized and sparsely distributed nature. To address this challenge, we propose a geometry regularized point cloud autoencoder, aiming to preserve local geometry structure. In particular, based on the Mahalanobis distance, we propose a point cloud geometry metric counting the local statistics. It endeavors to maximize the posterior of the reconstruction conditioned on the input point cloud. Our eigenspace analysis reveals the adaptivity of the developed metric---it behaves differently given different local structures. Moreover, a coarse-to-fine training strategy by varying the metric granularity is applied, leading to our proposed geometry regularized point cloud autoencoder. By applying our proposal to several off-the-shelf point cloud autoencoders, we show an improved point cloud reconstruction quality. In addition, the superior representability of our learned features is also demonstrated via an object classification task.