Documents
Research Manuscript
Supplementary - Towards Image Copy Detection at E-commerce Scale
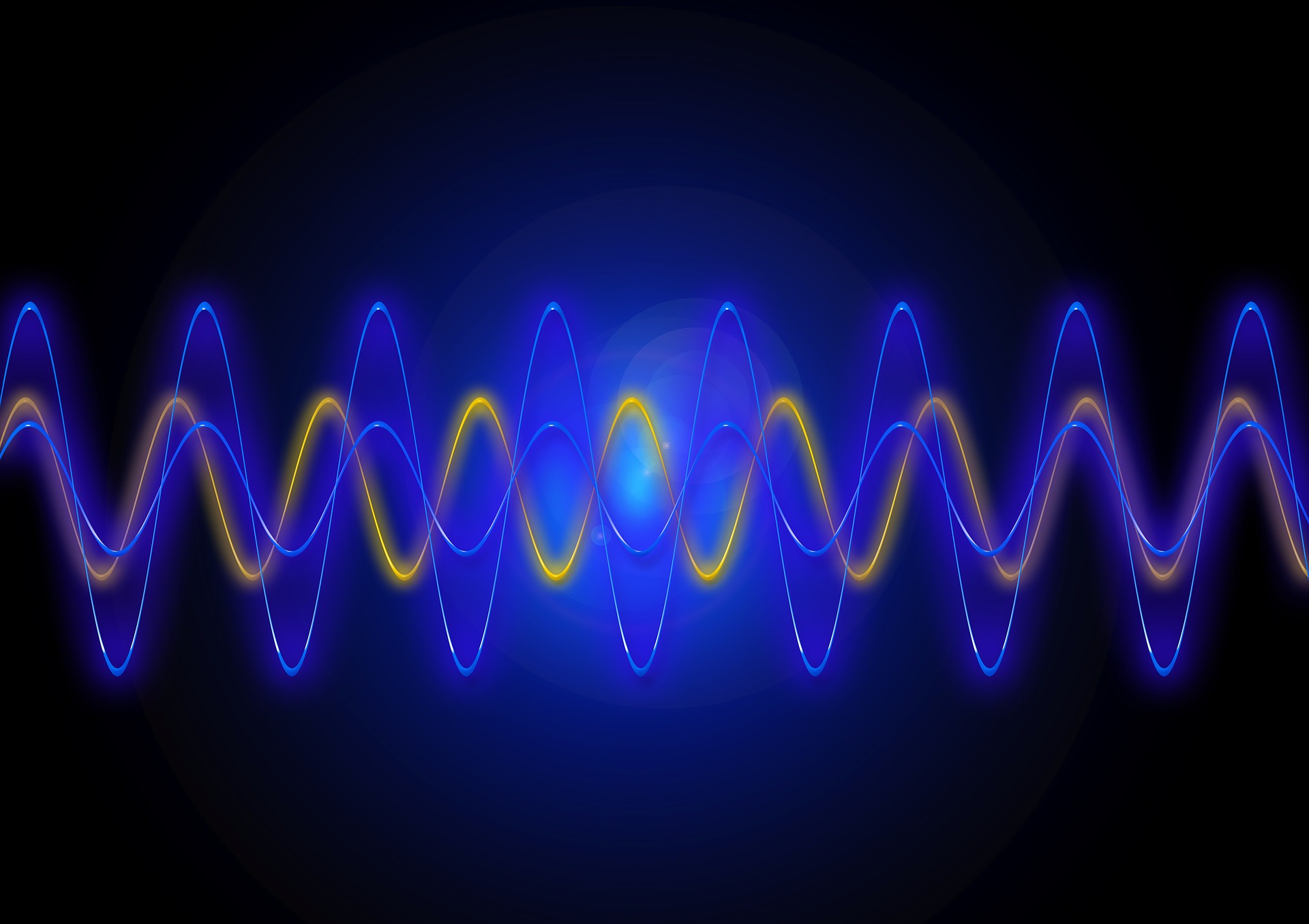
- Citation Author(s):
- Submitted by:
- Vishnu Suganth ...
- Last updated:
- 5 February 2025 - 5:34am
- Document Type:
- Research Manuscript
- Categories:
- Log in to post comments
Copy Detection system aims to identify if a query image is an edited/manipulated copy of an image from a large reference database with millions of images. While global image descriptors can retrieve visually similar images, they struggle to differentiate near-duplicates from semantically similar instances. We propose a dual-triplet metric learning (DTML) technique to learn global image features that group near-duplicates closer than visually similar images while maintaining the semantic structure of the embedding space. On the DISC2021 copy detection benchmark, DTML outperforms DINO and SSCD descriptors significantly. Additionally, we design an ensemble-based local feature matching strategy that operates on retrieved candidates to detect duplicates with high precision by effectively aggregating matched keypoints from individual methods. However, match scores from individual methods can be noisy and sensitive, making automated decisioning challenging. To address this, we develop a decisioning engine that consumes match scores from different keypoint detectors to make accurate decisions, demonstrating significant improvement over baselines across multiple datasets. We study the performance of our method under multiple datasets and demonstrate significant improvement over competitive baselines.