Documents
source code
Semantic Understanding of Vision Transformer Representation Spaces for Enhanced Medical Image Classification
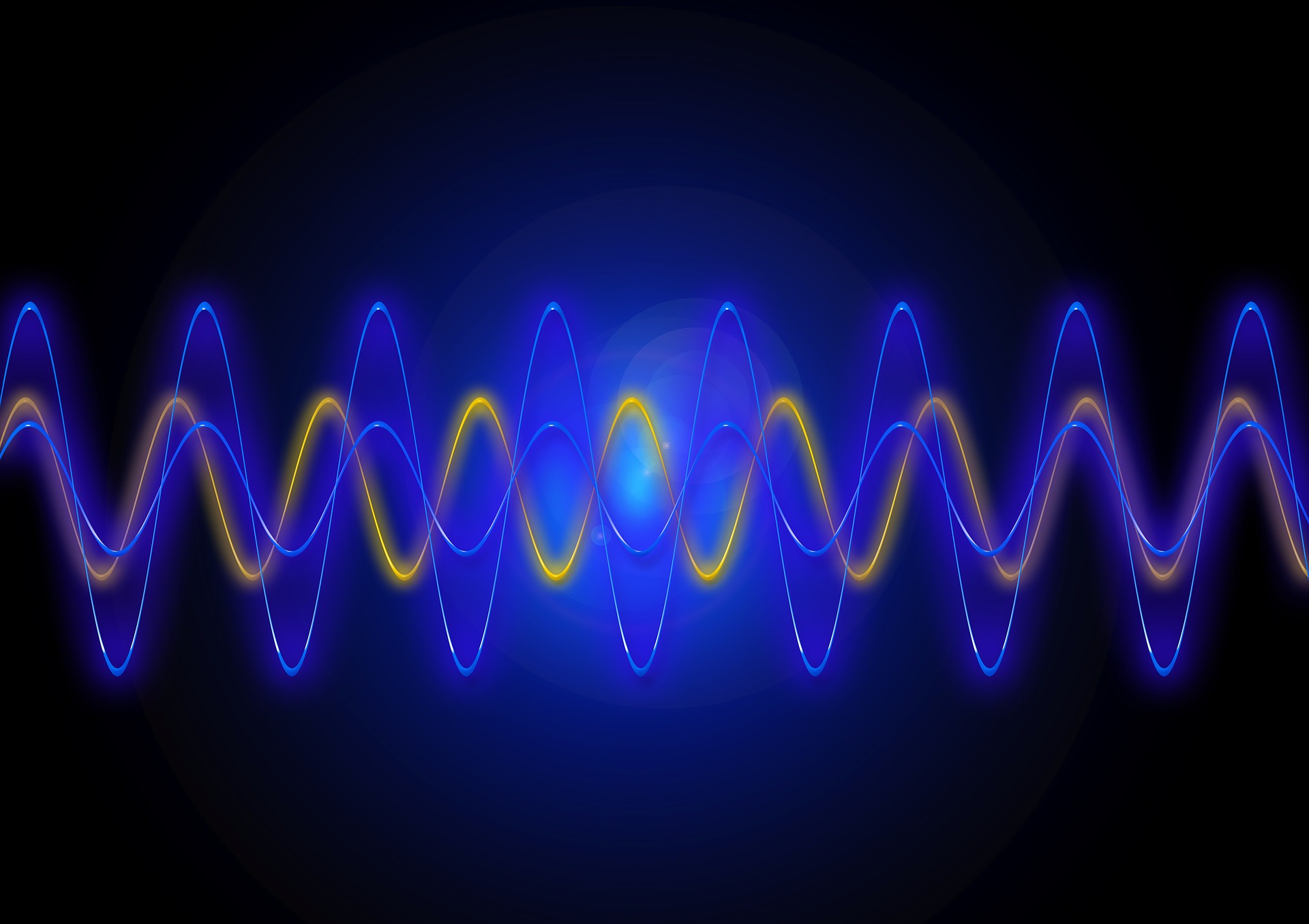
- Citation Author(s):
- Submitted by:
- MD Montasir Bin...
- Last updated:
- 6 February 2025 - 12:20am
- Document Type:
- source code
- Categories:
- Log in to post comments
In the last few years, vision transformers have increasingly been adopted for medical image classification and other applications due to their improved accuracies compared to other deep learning models. However, due to their size and complex interactions via the self-attention mechanism, they are not well understood. In particular, it is unclear whether the representations produced by such models are semantically meaningful. In this paper, using a projected gradient-based algorithm, we show that their representations are not semantically meaningful and they are inherently vulnerable to small changes. Images with imperceptible differences can have very different representations; on the other hand, images that should belong to different semantic classes can have nearly identical representations. Such vulnerabilities lead to unreliable classification results; for example, unnoticeable changes cause the classification accuracy to be reduced by over 60\%. To the best of our knowledge, this is the first work that studies the semantic meaningfulness of the representations from vision transformers for medical image classification.