Documents
Supplementary Material
Holistic Coreset Selection for Data Efficient Image Quality Assessment
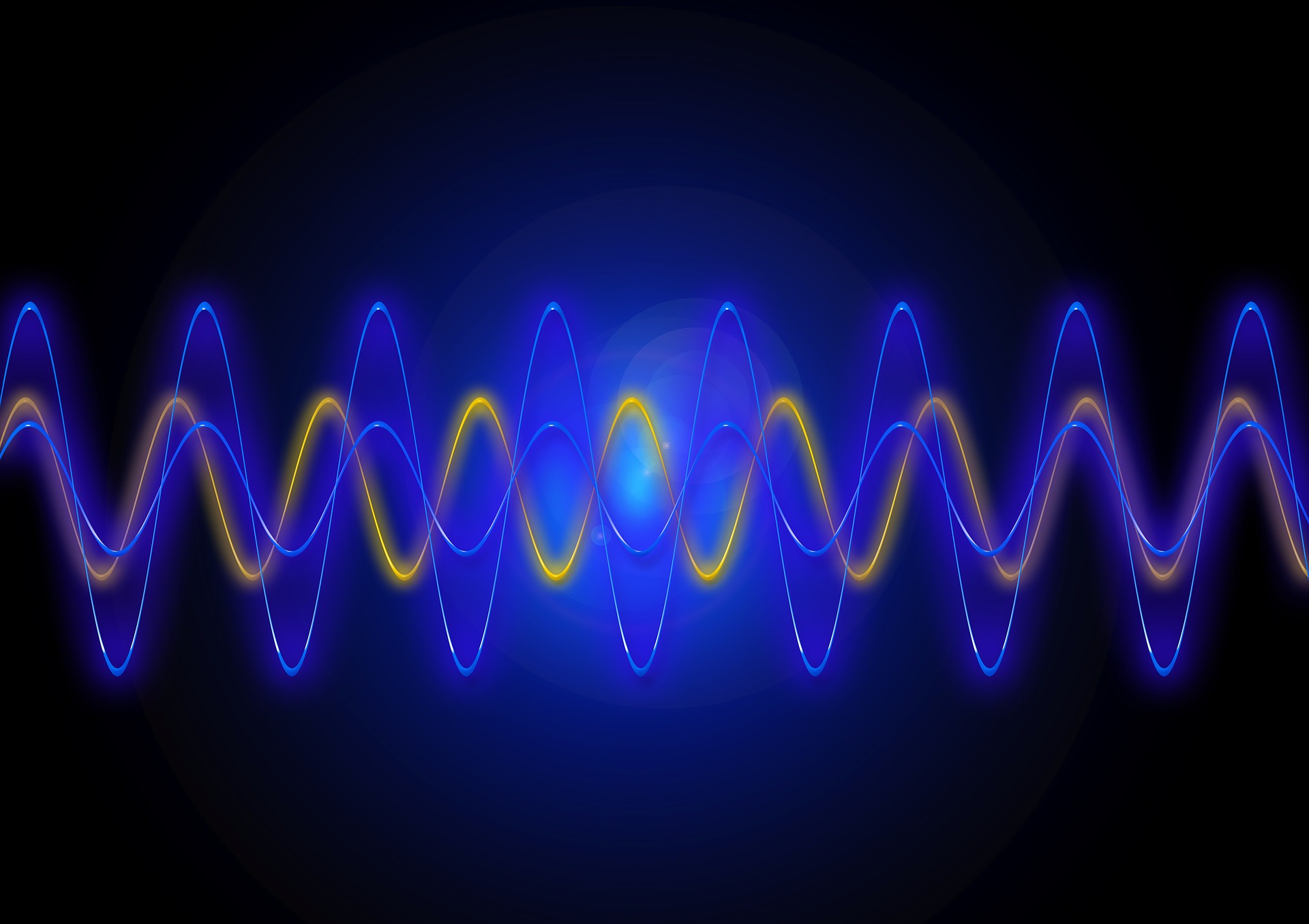
- DOI:
- 10.60864/m2w1-0x87
- Citation Author(s):
- Submitted by:
- Arpita Nema
- Last updated:
- 6 February 2025 - 5:47am
- Document Type:
- Supplementary Material
- Categories:
- Log in to post comments
Coreset selection improves training efficiency in deep learning but faces unique challenges in image quality assessment (IQA), where perceptual alignment with human vision and the interplay of distortions, semantics, and quality annotations are critical. Existing methods, designed for classification via static scoring criteria, fail to address IQA-specific multi-dimensional complexities, leading to suboptimal coreset construction. We propose perceptually guided coreset selection (PGCS), which employs adaptive sampling over a partitioned IQA latent space to dynamically balance distortion characteristics, perceptual quality, and semantic context. Latent space partitions are created based on the coverage of data points, ensuring that each partition reflects the overall structure of the dataset. Our adaptive sampling strategy dynamically adjusts selection range ensuring that local densities are preserved and better represented. Evaluated across five IQA benchmarks, PGCS-optimized coresets (5%-90% of original data) reduce dataset redundancy and annotation noise while achieving <=0.09 PLCC and <= 0.09 SRCC degradation with 5% data, matching or surpassing full-data training. Our work advances data-efficient learning by prioritizing perceptual alignment, offering a framework adaptable to human-centric vision tasks.
Comments
Supplementary Material
Submission!