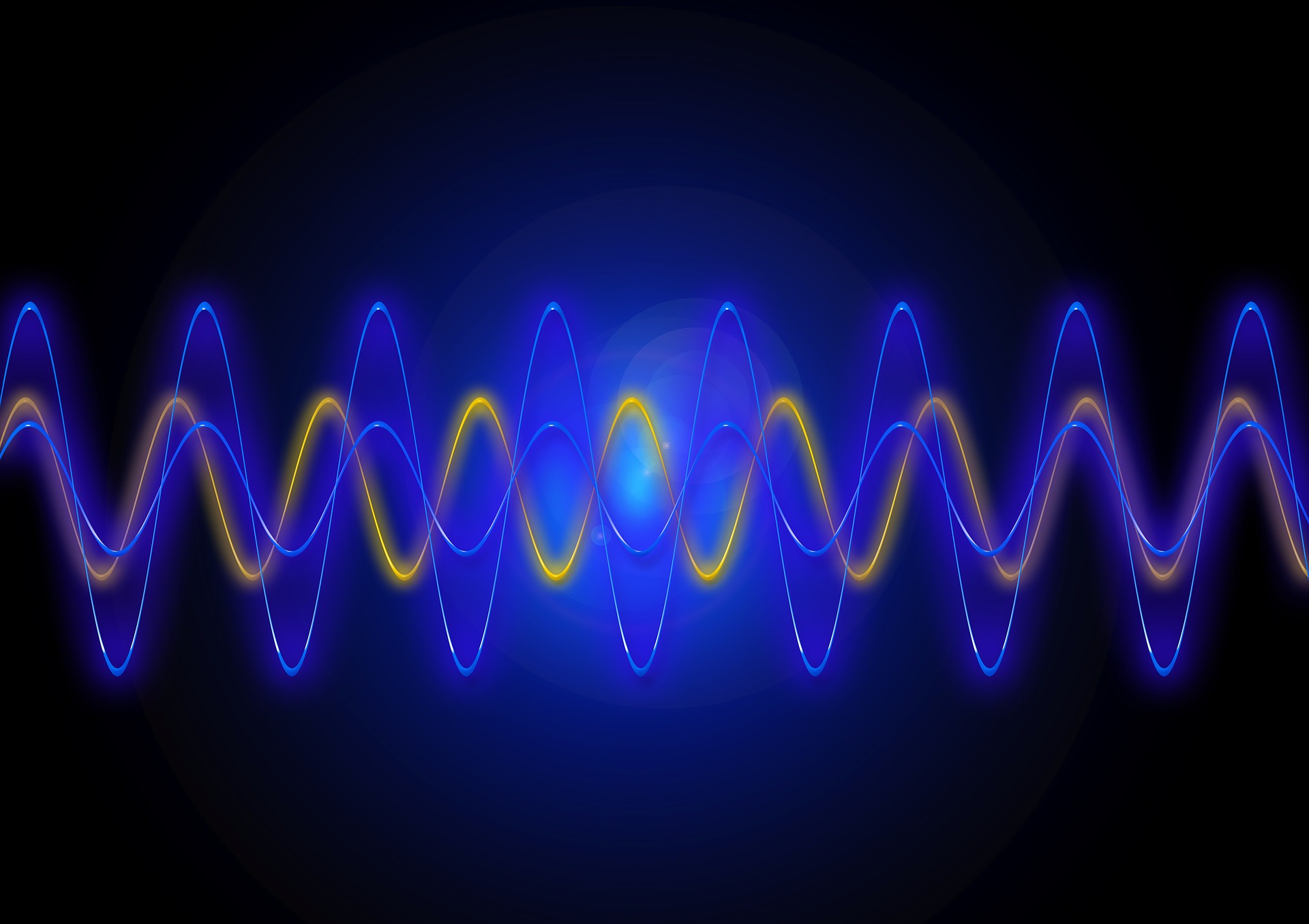
- Read more about Holistic Coreset Selection for Data Efficient Image Quality Assessment
- 1 comment
- Log in to post comments
Coreset selection improves training efficiency in deep learning but faces unique challenges in image quality assessment (IQA), where perceptual alignment with human vision and the interplay of distortions, semantics, and quality annotations are critical. Existing methods, designed for classification via static scoring criteria, fail to address IQA-specific multi-dimensional complexities, leading to suboptimal coreset construction.
- Categories:
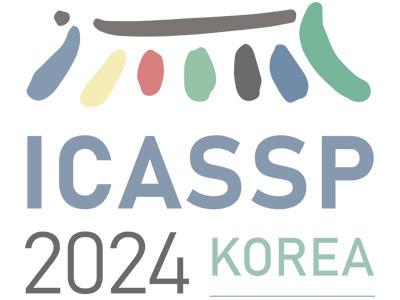
- Read more about KEEP_KNOWLEDGE_IN_PERCEPTION_slides
- Log in to post comments
This is the ppt of our paper: KEEP KNOWLEDGE IN PERCEPTION: ZERO-SHOT IMAGE AESTHETIC ASSESSMENT, in ICASSP 2024.
- Categories:
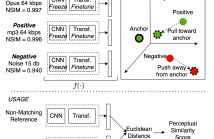
- Read more about NOMAD: Non-Matching Audio Distance
- Log in to post comments
This paper presents NOMAD (Non-Matching Audio Distance), a differentiable perceptual similarity metric that measures the distance of a degraded signal against non-matching references. The proposed method is based on learning deep feature embeddings via a triplet loss guided by the Neurogram Similarity Index Measure (NSIM) to capture degradation intensity. During inference, the similarity score between any two audio samples is computed through Euclidean distance of their embeddings. NOMAD is fully unsupervised and can be used in general perceptual audio tasks for audio analysis e.g.
- Categories:
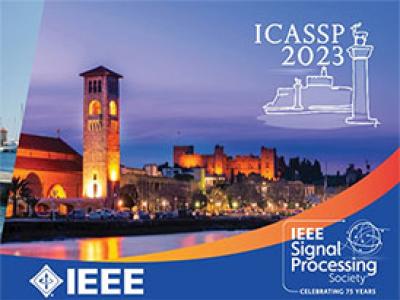
The short video has gained increasing attention in information sharing and commercial promotions due to the fast development of social platforms. Accompanying, it introduces great requirements for assessing the quality of short videos for efficient information acquirement and propagation. However, existing video quality assessment researches focus on assessing video content with five rating scores, limiting the assessment to a one-dimension and simplified criterion.
- Categories:
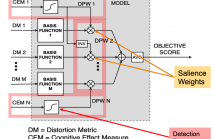
- Read more about A Data-driven Cognitive Salience Model for Objective Perceptual Audio Quality Assessment
- Log in to post comments
Objective audio quality assessment systems often use perceptual models to predict the subjective quality scores of processed signals, as reported in listening tests. Most systems map different metrics of perceived degradation into a single quality score predicting subjective quality. This requires a quality mapping stage that is informed by real listening test data using statistical learning (\iec a data-driven approach) with distortion metrics as input features.
- Categories:
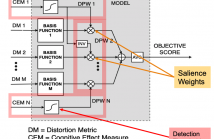
- Read more about A Data-Driven Cognitive Salience Model for Objective Perceptual Audio Quality Assessment
- Log in to post comments
Objective audio quality assessment systems often use perceptual models to predict the subjective quality scores of processed signals, as reported in listening tests. Most systems map different metrics of perceived degradation into a single quality score predicting subjective quality. This requires a quality mapping stage that is informed by real listening test data using statistical learning (\iec a data-driven approach) with distortion metrics as input features.
- Categories:
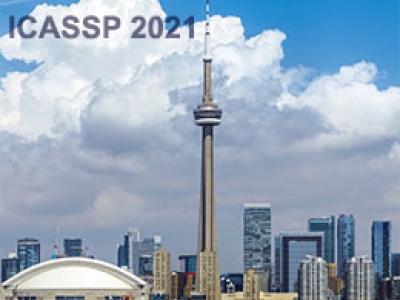
- Read more about AN END-TO-END NON-INTRUSIVE MODEL FOR SUBJECTIVE AND OBJECTIVE REAL-WORLD SPEECH ASSESSMENT USING A MULTI-TASK FRAMEWORK
- Log in to post comments
Speech assessment is crucial for many applications, but current intrusive methods cannot be used in real environments. Data-driven approaches have been proposed, but they use simulated speech materials or only estimate objective scores. In this paper, we propose a novel multi-task non-intrusive approach that is capable of simultaneously estimating both subjective and objective scores of real-world speech, to help facilitate learning. This approach enhances our prior work, which estimated subjective mean-opinion scores, where our
ICASSP Poster 3995.pdf

ICASSP Slides 3995.pdf

- Categories:
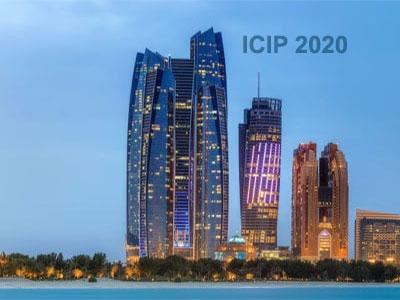
- Read more about A Comparative Evaluation of Temporal Pooling Methods for Blind Video Quality Assessment
- Log in to post comments
Many objective video quality assessment (VQA) algorithms include a key step of temporal pooling of frame-level quality scores. However, less attention has been paid to studying the relative efficiencies of different pooling methods on no-reference (blind) VQA. Here we conduct a large-scale comparative evaluation to assess the capabilities and limitations of multiple temporal pooling strategies on blind VQA of user-generated videos. The study yields insights and general guidance regarding the application and selection of temporal pooling models.
- Categories:
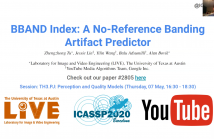
Banding artifact, or false contouring, is a common video compression impairment that tends to appear on large flat regions in encoded videos. These staircase-shaped color bands can be very noticeable in high-definition videos. Here we study this artifact, and propose a new distortion-specific no-reference video quality model for predicting banding artifacts, called the Blind BANding Detector (BBAND index). BBAND is inspired by human visual models. The proposed detector can generate a pixel-wise banding visibility map and output a banding severity score at both the frame and video levels.
- Categories:
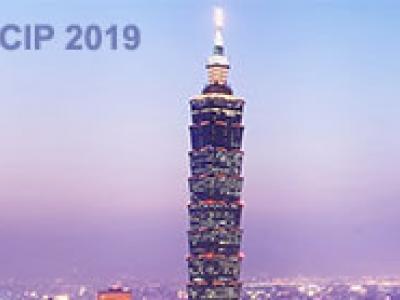
- Read more about A No-Reference Autoencoder Video Quality Metric
- Log in to post comments
In this work, we introduce the No-reference Autoencoder VidEo (NAVE) quality metric, which is based on a deep au-toencoder machine learning technique. The metric uses a set of spatial and temporal features to estimate the overall visual quality, taking advantage of the autoencoder ability to produce a better and more compact set of features. NAVE was tested on two databases: the UnB-AVQ database and the LiveNetflix-II database.
- Categories: