Documents
Supplementary Material
Supplementary Material
Multi Class Part Parsing based on Multi-class Boundaries ICIP 25 Supplementary Material
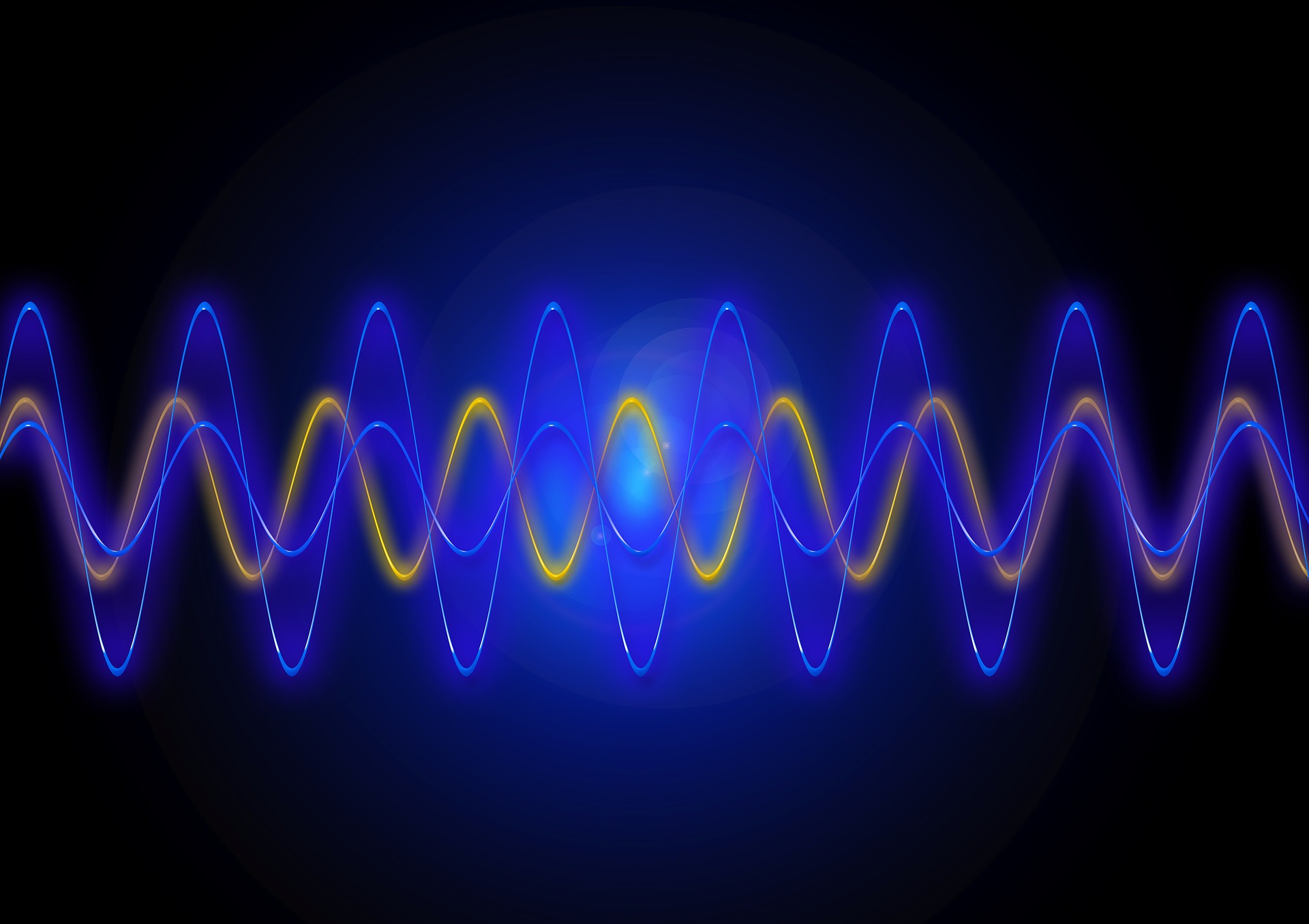
- DOI:
- 10.60864/7rxr-kh70
- Citation Author(s):
- Submitted by:
- Njuod Alsudays
- Last updated:
- 28 May 2025 - 7:35am
- Document Type:
- Supplementary Material
- Categories:
- Log in to post comments
Multi-class part parsing is a dense prediction task that segments objects into semantic components with multi-level abstractions. Despite its significance, this task remains challenging due to ambiguities at both part and class levels. In this paper, we propose a network that incorporates multi-class boundaries to precisely identify and emphasize the spatial boundaries of part classes, thereby improving segmentation quality. Additionally, we employ a weighted multi-label cross-entropy loss function to ensure balanced and effective learning from all parts. Experimental results validate the effectiveness of the proposed method, demonstrating its ability to enhance baseline performance on benchmark datasets.