Documents
Research Manuscript
ICIP2025_supp
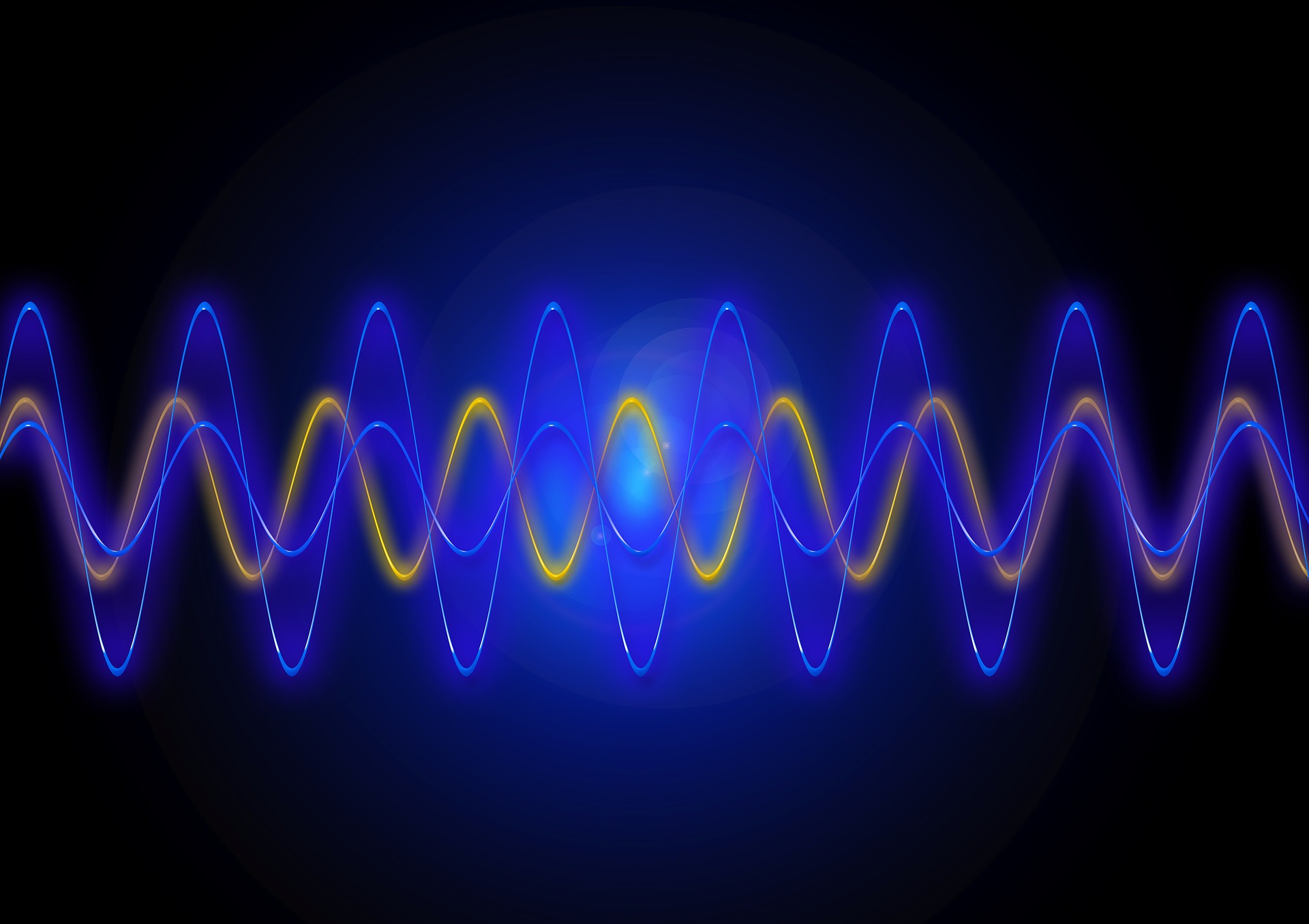
- DOI:
- 10.60864/c8jv-0y26
- Citation Author(s):
- Submitted by:
- Yuya Matsumoto
- Last updated:
- 6 February 2025 - 5:49am
- Document Type:
- Research Manuscript
- Categories:
- Log in to post comments
We address the challenge of local feature matching under large scale and rotation changes by focusing on keypoint positions.
First, we propose a novel module called similarity normalization (SN).
This module normalizes keypoint positions to remove a translation, rotation and scale difference between an image pair.
By performing positional encoding on these normalized positions, a network incorporated with SN can effectively avoid encoding largly different positions into descriptors from the two images.
Second, we apply strong data augmentation (DA) that includes large scale and rotation while existing matchers ignore such DA and are overfitted to upright image pairs.
In our experiments, SN and DA improves performance for image pairs with large scale and rotation.
In addition, the combination of SN with DA leads to additional performance improvements.