Documents
Description of Database/Benchmark
FACE QUALITY TRANSFORMER: A FACE QUALITY ASSESSMENT AND ENHANCEMENT FRAMEWORK
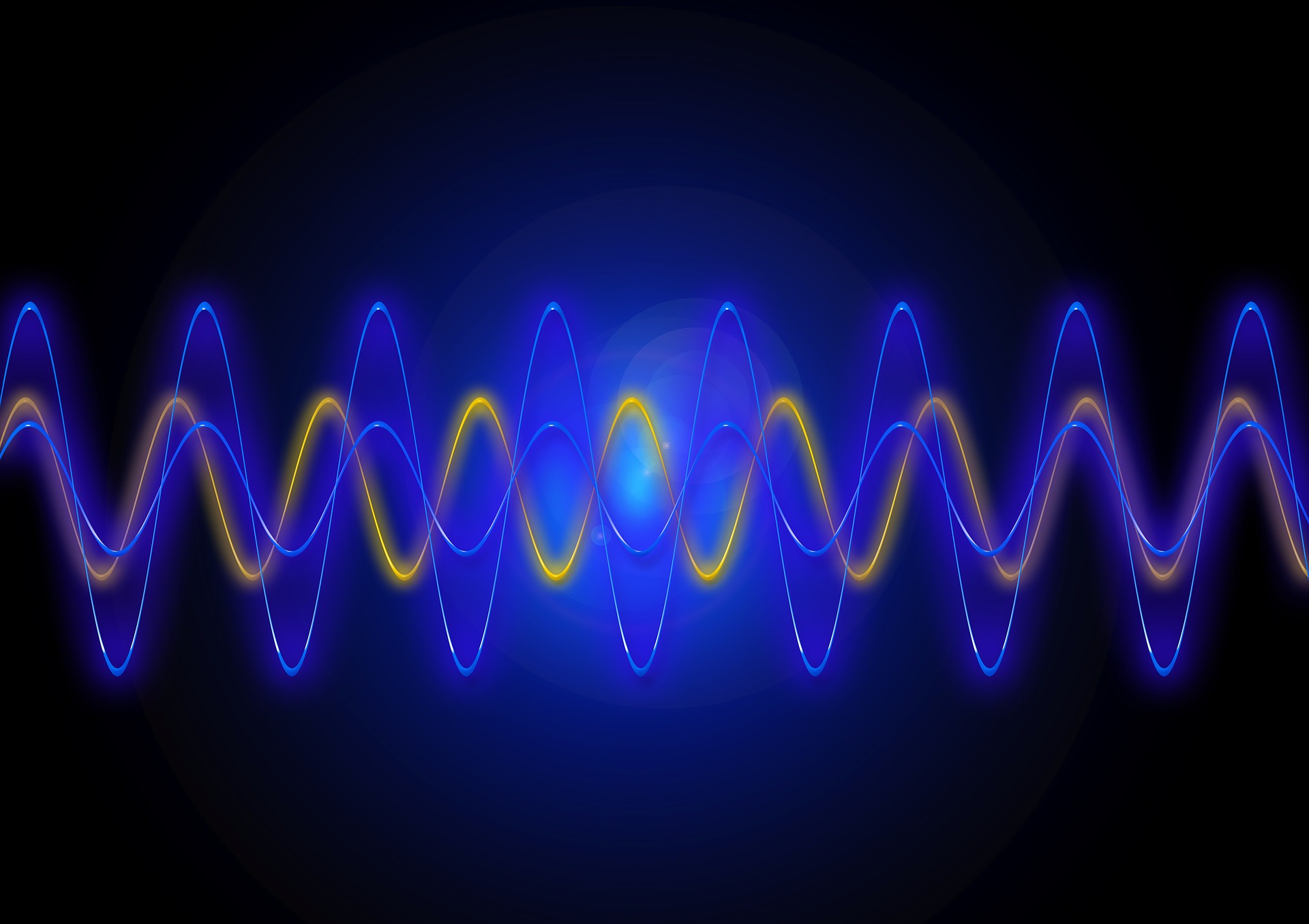
- Citation Author(s):
- Submitted by:
- Vishwajeet Shukla
- Last updated:
- 6 February 2025 - 6:32am
- Document Type:
- Description of Database/Benchmark
- Document Year:
- 2025
- Categories:
- Log in to post comments
The quality of image generation has reached impressive levels.
Advanced text-to-image models have become
amazingly good at creating objects, depicting actions with high precision.
However, despite significant progress in image generation,
the quality of generated faces remains a
critical factor for users. Even the most advanced text-to-image
diffusion models struggle to generate high quality faces consistently. This
highlights the importance of estimation of face quality in generated images as one of
the most important metric to assess. In this paper, we propose
a hybrid architecture comprising of attention-based Vision-Transformer
along with EfficientNet to capture intrinsic face
deformations present in image generation models. We also conduct a comparative
analysis of state-of-the-art diffusion models for face quality estimation including DALL-E, Flux, Stable Diffusion and Firefly.
Furthermore, we show that our proposed pipeline can be plugged with image generation
models to effectively correct the poor quality faces in generated images through automated re-generations.