Documents
Presentation Slides
Distributionally Robust Chance-Constrained Minimum Variance Beamforming
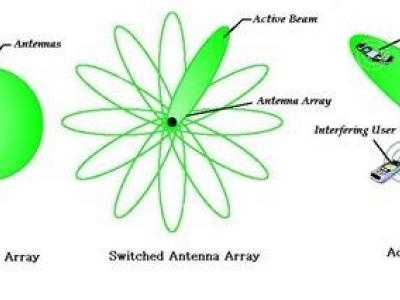
- Citation Author(s):
- Submitted by:
- Ning Ge
- Last updated:
- 23 March 2016 - 11:12am
- Document Type:
- Presentation Slides
- Document Year:
- 2016
- Event:
- Presenters:
- Xiao Zhang
- Paper Code:
- SAM-L1.2
- Categories:
- Keywords:
- Log in to post comments
This paper studies distributionally robust chance-constrained minimum variance beamforming. In contrast to deterministic modeling of the steering vector, our approach models the uncertainty statistically via distributions. We select the weights that minimize the combined output power subject to the distributionally robust chance constraint that for all distributions in the uncertainty set, the gain should exceed unity with high probability. Our discussion begins with the simplest case where the distributional set contains only Gaussian distribution; then we derive the robust weights for three distributional sets, namely, the set of (central symmetric) distributions with known mean and covariance, and a distributional model where the mean is known, the components are independent and belong to some known bounded intervals. It can be seen that these four robust beamformers provide statistical interpretation for the deterministic counterpart. Finally, we demonstrate the performance of these robust beamformers via several numerical examples.
Slides.pdf
