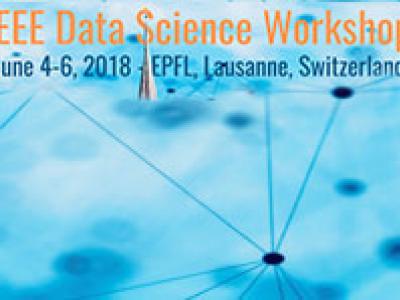
- Read more about Deep CNN Sparse Coding Analysis
- Log in to post comments
Deep Convolutional Sparse Coding (D-CSC) is a framework reminiscent
of deep convolutional neural nets (DCNN), but by omitting the learning of the
dictionaries one can more transparently analyse the role of the
activation function and its ability to recover activation paths
through the layers. Papyan, Romano, and Elad conducted an analysis of
such an architecture \cite{2016arXiv160708194P}, demonstrated the
relationship with DCNNs and proved conditions under which a D-CSC is
guaranteed to recover activation paths. A technical innovation of
- Categories: