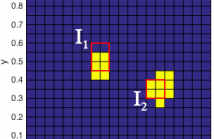
While convex optimization for low-light imaging has received some attention by the imaging community, non-convex optimization techniques for photon-limited imaging are still in their nascent stages. In this thesis, we developed a stage-based non-convex approach to recover high-resolution sparse signals from low-dimensional measurements corrupted by Poisson noise. We incorporate gradient-based information to construct a sequence of quadratic subproblems with an $\ell_p$-norm ($0 \leq p < 1$) penalty term to promote sparsity.
PhDForum.pdf

- Categories: