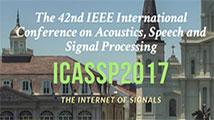
ICASSP is the world's largest and most comprehensive technical conference on signal processing and its applications. It provides a fantastic networking opportunity for like-minded professionals from around the world. ICASSP 2017 conference will feature world-class presentations by internationally renowned speakers and cutting-edge session topics. Visit ICASSP 2017
- Read more about ON DNN POSTERIOR PROBABILITY COMBINATION IN MULTI-STREAM SPEECH RECOGNITION FOR REVERBERANT ENVIRONMENTS
- Log in to post comments
A multi-stream framework with deep neural network (DNN) classifiers has been applied in this paper to improve automatic speech recognition (ASR) performance in environments with different reverberation characteristics. We propose a room parameter estimation model to determine the stream weights for DNN posterior probability combination with the aim of obtaining reliable log-likelihoods for decoding. The model is implemented by training a multi-layer
- Categories:
- Read more about HEARTMATE: AUTOMATED INTEGRATED ANOMALY ANALYSIS FOR EFFECTIVE REMOTE CARDIAC HEALTH MANAGEMENT
- Log in to post comments
Remote cardiac health management is an important healthcare application. We have developed Heartmate that enables basic screening of cardiac health using low cost sensors or smartphone-inbuilt sensors without manual intervention. It consists of robust denoising algorithm along with effective anomaly analytics for physiological signals. Heartmate identifies and eliminates signal corruption as well as detects cardiac anomaly condition from physiological cardiac signals like heart sound or phonocardiogram (PCG) and photoplethysmogram (PPG).
- Categories:
- Read more about RECONSTRUCTION OF 3D SURFACE FROM 2D HOLOGRAPHIC SIGNAL BASED ON KALMAN FILTER
- Log in to post comments
- Categories:
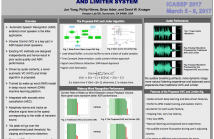
- Read more about DEEP LEARNING BASED AUTOMATIC VOLUME CONTROL AND LIMITER SYSTEM
- Log in to post comments
Automatic speech recognition is now playing an important role in volume control and adjustment of modern smart speakers. According to the recognition results by using the advanced deep neural network technology, this paper proposes an efficient processing system for automatic volume control (AVC) and limiter. The theoretical analyses, subjective and objective testing results show that the proposed processing system can offer a significant improvement for speech recognition performance during audio playback and improvement for audio playback performance in smart speakers.
- Categories:
- Read more about Fast Exemplar Selection Algorithm for Matrix Approximation and Representation: A Variant oASIS Algorithm
- Log in to post comments
Extracting inherent patterns from large data using decompositions of
data matrix by a sampled subset of exemplars has found many applications
in machine learning. We propose a computationally efficient
algorithm for adaptive exemplar sampling, called fast exemplar selection
(FES). The proposed algorithm can be seen as an efficient
variant of the oASIS algorithm (Patel et al). FES iteratively selects incoherent
exemplars based on the exemplars that are already sampled.
This is done by ensuring that the selected exemplars forms a positive
- Categories:
Group sparsity or nonlocal image representation has shown great potential in image denoising. However, most existing methods only consider the nonlocal self-similarity (NSS) prior of noisy input image, that is, the similar patches collected only from degraded input, which makes the quality of image denoising largely depend on the input itself. In this paper we propose a new prior model for image denoising, called group sparsity residual constraint (GSRC).
ICASSP--2017.pdf

- Categories:
- Read more about AUTOMATIC DETECTION OF SYLLABLE STRESS USING SONORITY BASED PROMINENCE FEATURES FOR PRONUNCIATION EVALUATION
- Log in to post comments
Automatic syllable stress detection is useful in assessing and diagnosing the quality of the pronunciation of second language (L2) learners in an automated way. Typically, the syllable stress depends on three prominence measures -- intensity level, duration, pitch -- around the sound unit with the highest sonority in the respective syllable. Stress detection is often formulated as a binary classification task using cues from the feature contours representing the prominence measures.
ICASSP17.pdf

- Categories:
- Read more about TWO-DIMENTIONAL ANTI-JAMMING COMMUNICATION BASED ON DEEP REINFORCEMENT LEARNING
- Log in to post comments
- Categories:
In this paper, we exploit deep convolutional features for object appearance modeling and propose a simple while effective deep iscriminative model (DDM) for visual tracking. The proposed DDM takes as input the deep features and outputs an object-background confidence map. Considering that both spatial information from lower convolutional layers and semantic information from higher layers benefit object tracking, we construct multiple deep discriminative models (DDMs) for each layer and combine these confidence maps from each layer to obtain the final object-background confidence map.
- Categories: