Documents
Presentation Slides
'MEDIA CLASSIFICATION WITH BAYESIAN OPTIMIZATION AND VAPNIK-CHERVONENKIS (VC) BOUNDS
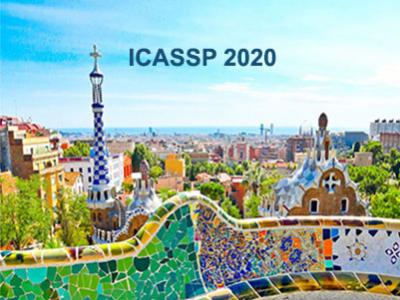
- Citation Author(s):
- Submitted by:
- Sunil Bharitkar
- Last updated:
- 13 May 2020 - 6:15pm
- Document Type:
- Presentation Slides
- Document Year:
- 2020
- Event:
- Presenters:
- Sunil Bharitkar
- Paper Code:
- MLSP-P8
- Categories:
- Log in to post comments
The automatic classification of content is an essential requirement for multimedia applications. Present research for audio-based classifiers uses short- and long-term analysis of signals, with temporal and spectral features. In our prior study, we presented an approach to classify streaming and local content, in real-time and with low latency, using synthetically-derived metadata features based on fixed class-conditional distributions. The three-class conditional distribution parameters were set a priori based on public information. In this paper, we extend the approach to optimizing the class condition distribution parameters, the neural network hyperparameters, and the size of the synthetic metadata training set using a combination of Bayesian optimization and by invoking the VC-dimensionality. We demonstrate that the resulting classifier is then able to improve classification accuracy by at least 10% with a modest increase in the computational complexity (in terms of multiply-adds) and decision time.
Comments
Presentation slides
Presentation slides