Documents
Presentation Slides
LEARNING GEOMETRIC FEATURES WITH DUAL-STREAM CNN FOR 3D ACTION RECOGNITION
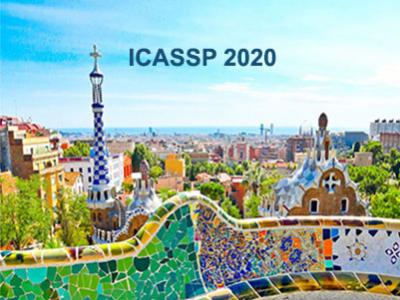
- Citation Author(s):
- Submitted by:
- Thien Huynh-The
- Last updated:
- 14 May 2020 - 10:53am
- Document Type:
- Presentation Slides
- Document Year:
- 2020
- Event:
- Presenters:
- Thien Huynh-The
- Paper Code:
- IVMSP-P5.5
- Categories:
- Log in to post comments
Recently, regarding several beneficial properties of depth camera, numerous 3D action recognition frameworks have studied high-level features by exploiting deep learning techniques, but nevertheless they cannot seize the meaningful characteristics of static human pose and dynamic action motion of a whole action. This paper introduces a deep network configured by two parallel streams of convolutional stacks for fully learning the deep intra-frame joint associations and inter-frame joint correlations, wherein the structure of each stream is learned from Inception-v3. In experiments, besides the compatibility verification with various backbone networks, the proposed approach achieves state-of-the-art performance in battle with several deep learning-based methods on the updated NTU RGB+D 120 dataset.
Comments
Q&A
Question: What is network parameters the proposed method, compared with existing methods (in Table of Method Comparison in the slide)?
Answer: Thank for your interesting question. In this paper, we introduce a framework of dual-stream CNN for 3D human action recognition, in which each stream can be the transfer learning from a CNN backbone. For example, in the Table, we show the accuracy with different networks, such as GoogleNet, ResNet, and DenseNet. The network parameter includes the weights and bias of kernels in the convolutional layers and of hidden nodes in the fully connected layers. Following the network architecture of CNNs in the comparison, VGG-19 is the heaviest one. We hope that our answer can satisfy your query.