Documents
Poster
CONCSS: CONTRASTIVE-BASED CONTEXT COMPREHENSION FOR DIALOGUE-APPROPRIATE PROSODY IN CONVERSATIONAL SPEECH SYNTHESIS
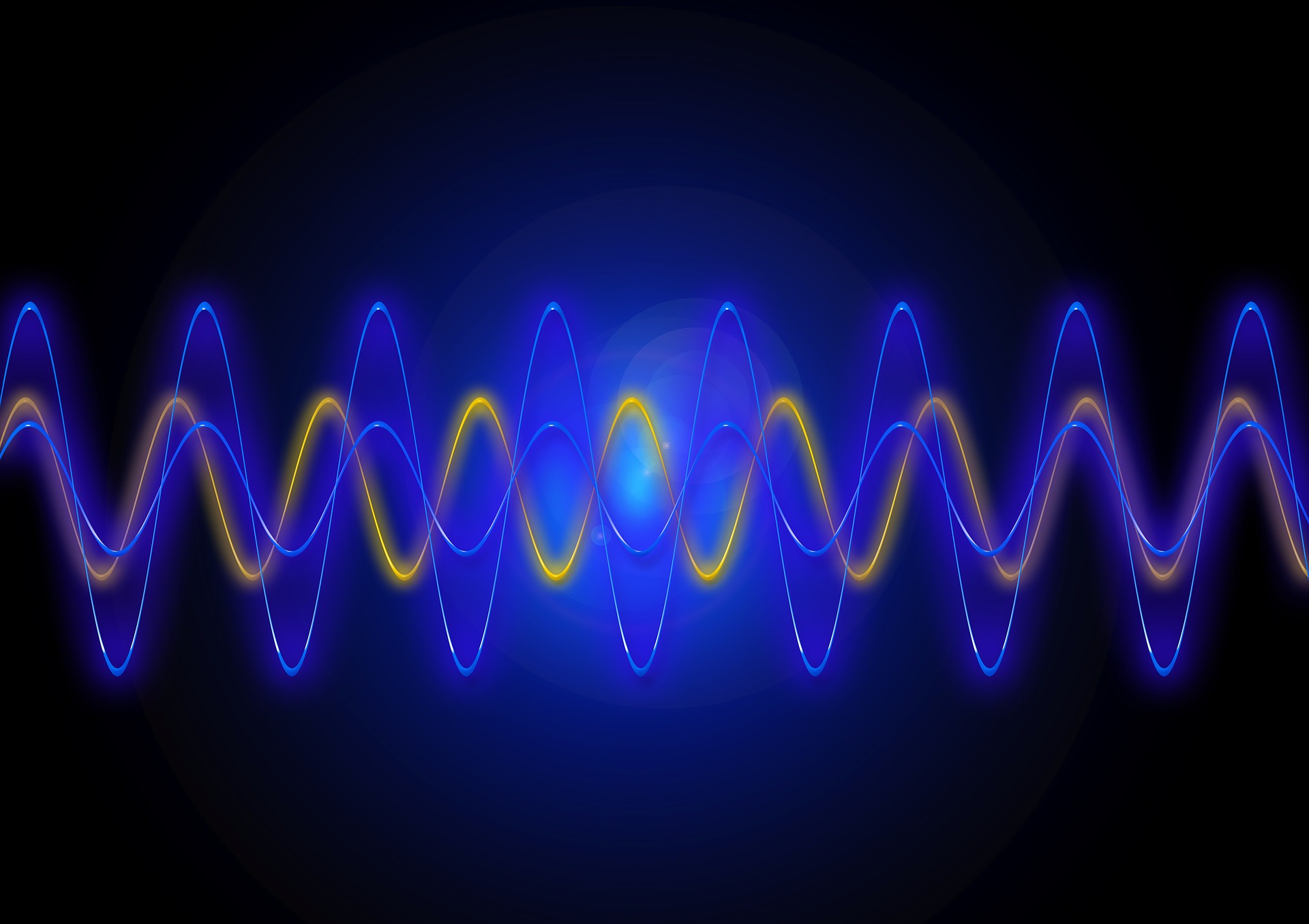
- DOI:
- 10.60864/xa1h-dj67
- Citation Author(s):
- Submitted by:
- Yayue Deng
- Last updated:
- 6 June 2024 - 10:28am
- Document Type:
- Poster
- Categories:
- Log in to post comments
Conversational speech synthesis (CSS) incorporates historical dialogue as supplementary information with the aim of generating speech that has dialogue-appropriate prosody. While previous methods have already delved into enhancing context comprehension, context representation still lacks effective representation capabilities and context-sensitive discriminability. In this paper, we introduce a contrastive learning-based CSS framework, CONCSS. Within this framework, we define an innovative pretext task specific to CSS that enables the model to perform self-supervised learning on unlabeled conversational datasets to boost the model's context understanding. Additionally, we introduce a sampling strategy for negative sample augmentation to enhance context vectors' discriminability. This is the first attempt to integrate contrastive learning into CSS. We conduct ablation studies on different contrastive learning strategies and comprehensive experiments in comparison with prior CSS systems. Results demonstrate that the synthesized speech from our proposed method exhibits more contextually appropriate and sensitive prosody.
Comments
The poster
The poster