Documents
Presentation Slides
Multimodal-Enhanced Objectness Learner for Corner Case Detection in Autonomous Driving
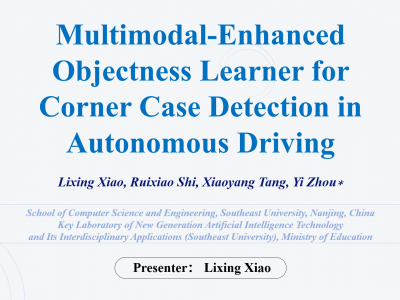
- DOI:
- 10.60864/kdhg-3a23
- Citation Author(s):
- Submitted by:
- Lixing Xiao
- Last updated:
- 8 November 2024 - 8:37pm
- Document Type:
- Presentation Slides
- Document Year:
- 2024
- Event:
- Presenters:
- Lixing Xiao
- Paper Code:
- https://github.com/tryhiseyyysum/MENOL
- Categories:
- Log in to post comments
Previous works on object detection have achieved high accuracy in closed-set scenarios, but their performance in open-world scenarios is not satisfactory. One of the challenging open-world problems is corner case detection in autonomous driving. Existing detectors struggle with these cases, relying heavily on visual appearance and exhibiting poor generalization ability. In this paper, we propose a solution by reducing the discrepancy between known and unknown classes and introduce a multimodal-enhanced objectness notion learner. Leveraging both vision-centric and image-text modalities, our semi-supervised learning framework imparts objectness knowledge to the student model, enabling class-aware detection. Our approach, Multimodal-Enhanced Objectness Learner (MENOL) for Corner Case Detection, significantly improves recall for novel classes with lower training costs. By achieving a 76.6% mAR-corner and 79.8% mAR-agnostic on the CODA-val dataset with just 5100 labeled training images, MENOL outperforms the baseline ORE by 71.3% and 60.6%, respectively.
Comments
Added Oral Presentation
Added Oral Presentation slides in ICIP2024 and the related code link on Github.