Documents
Poster
1-D Spatial Attention in Binarized Convolutional Neural Networks
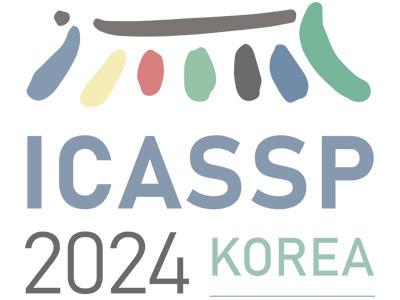
- DOI:
- 10.60864/c5te-1187
- Citation Author(s):
- Submitted by:
- WANSOO KIM
- Last updated:
- 7 April 2024 - 9:12pm
- Document Type:
- Poster
- Document Year:
- 2024
- Event:
- Paper Code:
- MLSP-P21.9
- Categories:
- Log in to post comments
This paper proposes a structure called SPBNet for enhancing binarized convolutional neural networks (BCNNs) using a low-cost 1-D spatial attention structure. Attention blocks can compensate for the performance drop in BCNNs. However, the hardware overhead of complex attention blocks can be a significant burden in BCNNs. The proposed attention block consists of low-cost 1-D height-wise and width-wise 1-D convolutions, It has the attention bias to adjust the effects of attended features in ×0.5 − ×1.5. In experiments, the proposed block used in ResNet18-based BCNNs improves Top-1 accuracy up to 2.7% over a baseline ReActNet on the CIFAR- 100 dataset. Notably, without using teacher-student training, the proposed structure can show comparable performance as the baseline ReActNetA using teacher-student training.
Comments
GitHub repository link
https://github.com/EmPasLab/SPBNet