Documents
Poster
Accurate Dictionary Learning with Direct Sparsity Control
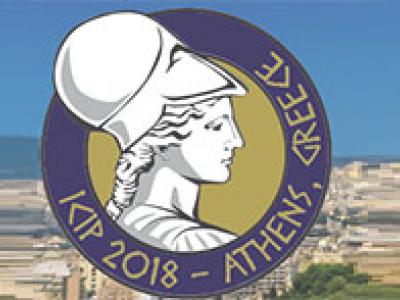
- Citation Author(s):
- Submitted by:
- Adrian Barbu
- Last updated:
- 5 October 2018 - 6:03am
- Document Type:
- Poster
- Document Year:
- 2018
- Event:
- Presenters:
- Adrian Barbu
- Paper Code:
- MQ.P4.7
- Categories:
- Log in to post comments
Dictionary learning is a popular method for obtaining sparse linear representations for high dimensional data, with many applications in image classification, signal processing and machine learning.
In this paper, we introduce a novel dictionary learning method based on a recent variable selection algorithm called Feature Selection with Annealing (FSA). Because FSA uses an L0 constraint instead of the L1 penalty, it does not introduce any bias in the coefficients and obtains a more accurate sparse representation. Furthermore, the L0 constraint makes it easy to directly specify the desired sparsity level instead of indirectly through a L1 penalty. Finally, experimental validation on real gray-scale images shows that the proposed method obtains higher accuracy and efficiency in dictionary learning compared to classical methods based on the L1 penalty.
poster.pdf
