Documents
Poster
Active selection of source patients in transfer learning for epileptic seizure detection using Riemannian Manifold
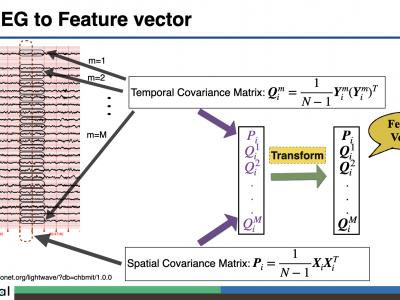
- Citation Author(s):
- Submitted by:
- Kazi Mahmudul Hassan
- Last updated:
- 28 May 2023 - 8:15am
- Document Type:
- Poster
- Document Year:
- 2023
- Event:
- Presenters:
- KAZI MAHMUDUL HASSAN
- Paper Code:
- 5105
- Categories:
- Log in to post comments
Epileptic seizure detection from long recordings of scalp electroencephalography (EEG) is a challenging task owing to their unpredictability in nature with the inclusion of noise, artifacts and subject dependency. We hypothesize that selection of training EEG data plays important role in the model performance. Thus, we introduced an active learning based training data selection and modification method with a Riemannian geometry, centroid alignment, tangent space mapping and a support vector machine classifier. The proposed method focuses on the strategy of a patient-specific training approach with a patient-independent evaluation. Both the spatial and temporal correlations between and within the EEG channels are taken into the consideration. The benchmark CHB-MIT dataset is used to evaluate our model. For our model, we reported an average sensitivity of 86.8%, specificity of 85.4%, and accuracy of 86.1% with a receiver operating characteristics-area under the curve (ROC-AUC) of 93.1%, which has encouraged us to further improve its performance.