Documents
Presentation Slides
An Adaptive Multi-Scale and Multi-Level Features Fusion Network with Perceptual Loss for Change Detection
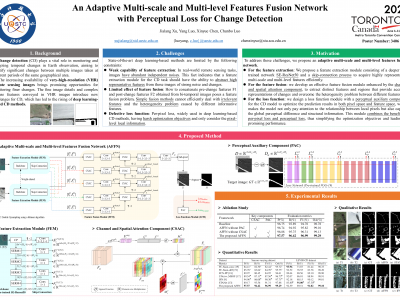
- Citation Author(s):
- Submitted by:
- Jialang Xu
- Last updated:
- 22 June 2021 - 1:49am
- Document Type:
- Presentation Slides
- Document Year:
- 2021
- Event:
- Presenters:
- Jialang Xu
- Paper Code:
- IVMSP-27.5
- Categories:
- Log in to post comments
Change detection plays a vital role in monitoring and analyzing temporal changes in Earth observation tasks. This paper proposes a novel adaptive multi-scale and multi-level features fusion network for change detection in very-high-resolution bi-temporal remote sensing images. The proposed approach has three advantages. Firstly, it excels in abstracting high-level representations empowered by a highly effective feature extraction module. Secondly, an elaborate feature fusion module incorporated with the channel and spatial attention mechanism is proposed to provide efficient fusion strategies for multi-scale and multi-level features from bi-temporal images and multiple convolutional layers. Finally, a novel perceptual auxiliary component is designed to capture the perceptual loss of the global perceptual and structural differences and address the optimization problem caused by only using per-pixel loss function in change detection. Comprehensive experiments on two benchmark datasets confirm that our proposed framework outperforms state-of-the-art algorithms in both quantitative assessment and visual interpretation.
MFPNet_poster.pdf
